AI-driven combined deformable registration and image synthesis between radiology and histopathology
PO-1613
Abstract
AI-driven combined deformable registration and image synthesis between radiology and histopathology
Authors: Amaury Leroy1,2, Marvin Lerousseau2, Théophraste Henry2, Théo Estienne2, Marion Classe3,2, Nikos Paragios1, Eric Deutsch2, Vincent Grégoire4
1Therapanacea, Artificial Intelligence, Paris, France; 2Gustave Roussy, Paris-Saclay University, Inserm 1030, Molecular Radiotherapy and Therapeutic Innovation, Villejuif, France; 3Gustave Roussy, Pathology Department, Villejuif, France; 4Centre Léon Bérard, Radiation Oncology Department, Lyon, France
Show Affiliations
Hide Affiliations
Purpose or Objective
Although widely used for all steps of cancer treatment,
radiologic imaging modalities (CT, MRI, …) provide insufficient assessment of
tissue properties and cancer proliferation. A complete understanding of tumor
micro-environment often goes through additional pathologic examination on
surgically excised specimens requiring a multimodal registration between 2D
histological slide and 3D anatomical volume. Yet, this step is substantially
difficult because of the extreme shrinkage and out-of-plane deformations that
the tissue undergoes through histological process, the differences in
resolution scales and color intensities, often imposing a burdensome
time-consuming manual mapping. The aim of our work is to provide an end-to-end
deep learning framework to automatically register 2D histopathology with 3D
radiology in an unsupervised and deformable setting. The latter could directly
be integrated into the treatment plan for better delineation and
comprehension of tumor heterogeneity towards dose painting.
Material and Methods
We have settled a private cohort made of 75 patients
(45/10/20 for train/validation/test sets) on whom were acquired both
pre-operative H&N CT scan and digitalized whole slide images after total
laryngectomy. The number of histopathology slides per patient ranges from 4 to
11 with a theoretical spacing of at least 5mm between them. Our work’s novelty is
two-fold: first, to solve the multimodal issue, we developed a generative
framework based on cycleGANs that predict CT from histology and vice versa.
Second, concerning the dimensional constraint, the resulting 2D synthetic CT
along with the original 3D CT become the input for a multi-slices-to-volume
registration pipeline in a fully unsupervised learning context. End-to-end
integration allows a direct mapping CT/histology and provides the radiation
oncologist with additional biological insights.
Results
Figure 1 presents the pipeline with one example from the
test set. For the GAN-based generative pipeline, we report a Structure
Similarity index of 0.78 (1 = perfect reconstruction). The 2D-3D registration
model enables direct pixel-wise mapping between histology and CT scan.
Visually, the deformed CT looks very close to the original histology, and we
computed normalized mutual information of 0.89 on the masked predictions (1 =
perfect correlation). Annotations on both modalities (tumor, OARs) are in
progress and will soon assess the functional quality of the registration
through metrics like Dice score.
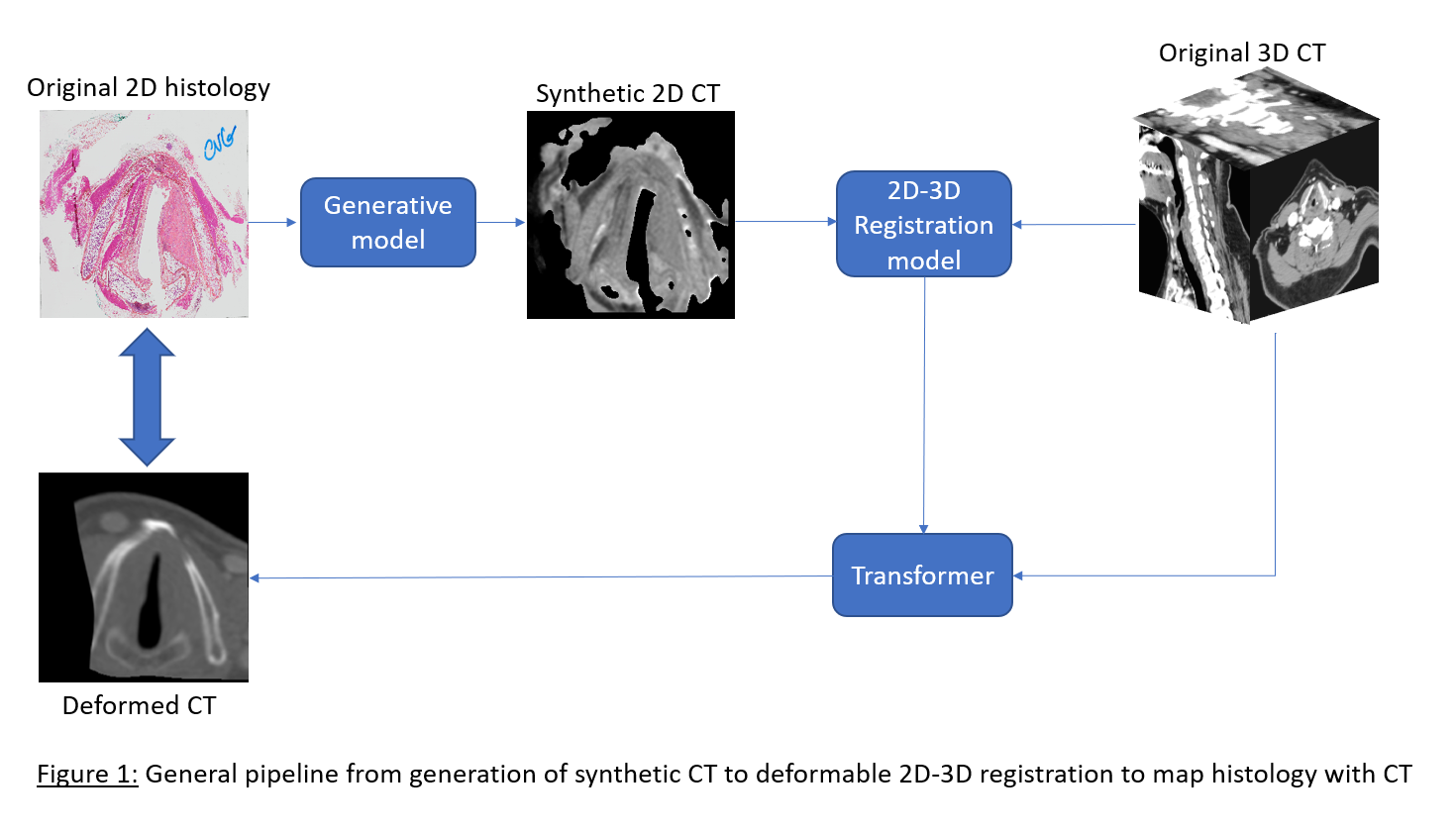
Conclusion
To our knowledge, this framework is the first to automatically
register radiology and histopathology in a deformable and 2D-3D setting. The
same model can be applied to other anatomical imaging like MR and will enable pixel-wise
comparison of tumor annotations with gold standard histology. Next, meaningful
biological signatures from anatomical modalities will straightforward be
extracted. Finally, it is one more step towards in-vivo virtual histology that
could be a game-changer in oncology.