Slice-by-slice deep learning aided oropharyngeal cancer segmentation on PET and CT images
Alessia De Biase,
The Netherlands
PO-1606
Abstract
Slice-by-slice deep learning aided oropharyngeal cancer segmentation on PET and CT images
Authors: Alessia De Biase1, Nanna M. Sijtsema1, Johannes A. Langendijk1, Lisanne V. van Dijk1, Peter M.A. van Ooijen2,3
1University Medical Centre Groningen (UMCG), Radiotherapy, Groningen, The Netherlands; 2University Medical Centre Groningen (UMCG), Radiology, Groningen, The Netherlands; 3University Medical Centre Groningen (UMCG), Data Science Centre in Health (DASH), Groningen, The Netherlands
Show Affiliations
Hide Affiliations
Purpose or Objective
Tumour segmentation is a fundamental step for
radiotherapy treatment planning. To define an accurate segmentation of the
primary tumour (GTVp) of OPC patients, simultaneous assessment of different
image modalities is needed. Each image volume is explored slice-by-slice from
different orientations, resulting in a tedious and time consuming process. Moreover,
the manual fixed boundary of each segmentation neglects the spatial uncertainty
known to occur in tumour delineation. This study aims to assist radiation
oncologists in a slice-by-slice adaptive GTVp segmentation using probability
maps, proposing a novel automatic deep learning (DL) segmentation model, on
registered PET-CT images.
Material and Methods
Based on the inclusion criteria 105 OPC patients
treated with (chemo)radiation between 2014 and 2017 in our institute were
included. PET and CT images and GTVp contours, used for radiotherapy treatment
planning, were collected. PET and CT images were registered rigidly. Bounding
boxes of 144×144×144mm3 were extracted around the oropharynx. An external
validation set of 200 patients from 4 different centres was used. The DL
framework was built in order to perform segmentation utilizing both inter and
intra-slice context. The model was trained on sequences of 3 consecutive 2D
slices of concatenated PET and CT images, while the GTVp contours are used as
ground truth. A 5-fold cross validation was performed three times, training on
sequences extracted from the Axial (A), Sagittal (S) and Coronal (C) plane,
respectively. The Dice Score Coefficient (DSC) was used to select the best
model in each fold. In the testing phase, each slice resulted in three
predictions (except for slices at the boundaries of the volume) that were
averaged, creating three final segmentation outputs for the A,S and C planes.
Results
Table 1 reports quantitative results that measure
the quality of the proposed model on PET-CT images. The framework has higher
performance on the A and S planes compared to the C plane. The model trained on
the C planes resulted in more false positives in slices without tumour. Figure 1
shows an example of a probability map obtained by the proposed slice-by-slice
segmentation method. The network uses the knowledge gained by the previous and
the successive slice, resulting in areas with different probabilities of
predicting tumour.
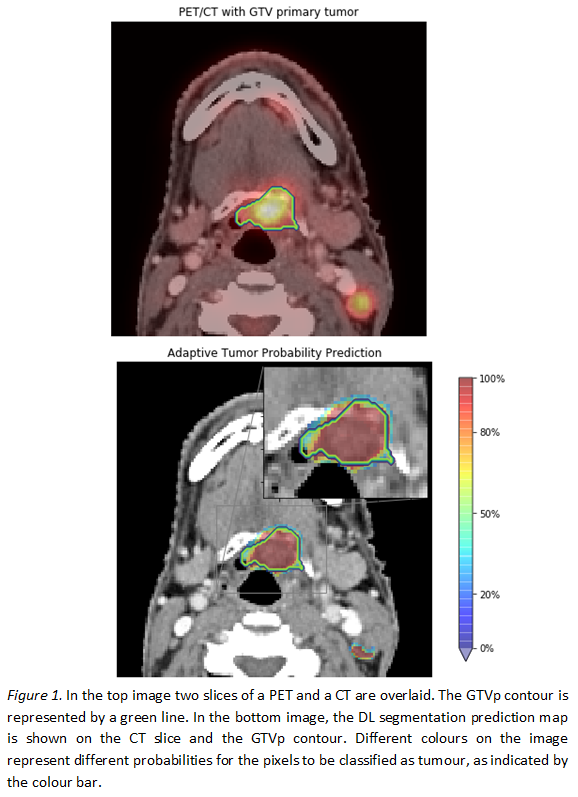
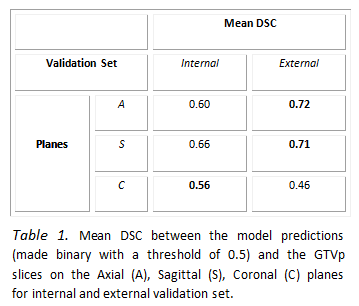
Conclusion
Since the GTVp is used as ground truth, the quality
of the contours highly affects the performance of the proposed model and the
evaluation of results. The lower performance on the internal validation set, on
the A and S planes, could be explained by a larger variability in GTVp contours
in training set than in the external set. The bony structure and the metal
artefacts on the CT images seem to be misleading for tumour classification on
the C plane. The results from the proposed novel DL segmentation model are
promising. The probability maps, on registered PET-CT images, can guide
radiation oncologists in a slice-by-slice adaptive GTVp segmentation.