Detection of mandibular osteoradionecrosis using novel imaging biomarkers for head and neck cancer
PO-1779
Abstract
Detection of mandibular osteoradionecrosis using novel imaging biomarkers for head and neck cancer
Authors: Abdallah Mohamed1, Abdulrahman Abusaif1, Ahmed Moawad2, Lisanne van Dijk1, David Fuentes3, Khaled Elsayes4, Clifton Fuller5, Stephen Lai6
1MD Anderson Cancer Center, Radiation Oncology, Houston, USA; 2MD Anderson Cancer Center, Diagnostic Imaging, Houston, USA; 3MD Anderson Cancer Center, Imaging Physics, Houston, USA; 4MD Anderson Cancer Center, Diagnostic Imaging, Houston, USA; 5MD Anderson Cancer Center, Radiation Oncology, Houston, USA; 6MD Anderson Cancer Center, Head and Neck Surgery, Houston, USA
Show Affiliations
Hide Affiliations
Purpose or Objective
This work aims to identify imaging biomarkers to early detect osteoradionecrosis (ORN) in head and neck cancer patients after radiation treatment (RT).
Material and Methods
This
retrospective study was approved by the institutional review board. We
identified patients with confirmed ORN diagnosis at MD Anderson Cancer Center
between 2008 and 2018. We retrieved all the post-RT contrast-enhanced CT scans
(CECTs) of these patients and selected the first study after the ORN diagnosis.
First, we manually segmented the mandibular ORN volumes in all studies using a
minimalistic approach (i.e. the least volume possible was segmented without including
“a safety margin” of normal bone) to ensure that the image region defined by
the mask contains textural features of ORN only. Then, control normal
mandibular volumes were created on the contralateral healthy mandible. The
segmentations on the CECTs of both ORN and control masks were used to extract the
radiomic features using the PyRadiomics® platform version 2.1.1 after the application of intrinsic filters.
Redundant radiomic features that are highly correlated with other features were
removed when pairwise correlation≥0.99. Subsequently, filter
algorithms were used to further reduce the number of radiomic features. After
that, wrapper and embedded methods were applied on the resulting radiomic
features. Gini importance and Recursive Feature Elimination (RFE) were used to
select the final radiomic features for the predictive model. Internal
validation of the classifier was done using 5-folds cross-validation (CV-5). The
performance of the model was evaluated using Area Under Curve (AUC) of the Receiver
Operator Curve (ROC). The workflow is detailed in Figure 1.
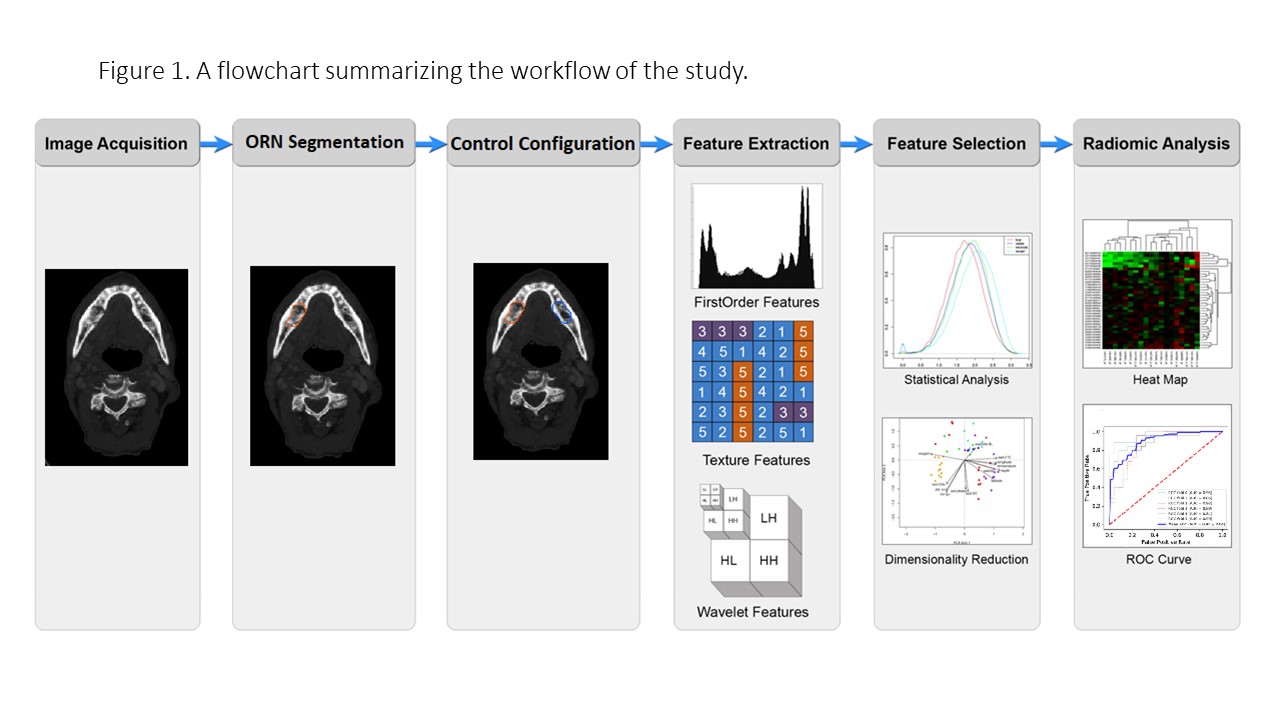
Results
A total of 150 patients with radiologically established ORN were
included in our study. The mean age was 62.3 years (range 27-82). The mean duration between the end of RT
and ORN diagnosis was 32.6 months. The
pairwise correlation omitted 432 features with a correlation ≥ 0.99. After
that, the first step of the radiomic features engineering (using the filter
algorithm) resulted in the selection of 33 radiomic features with statistically
significant results in all the following three statistical methods: Pearson
correlation, Chi-square test, and F-score. The RFE based on the Gini index selected
5 radiomics features. The
final classifier used SVM with linear Kernel. The input for this classifier was
the final set of radiomic features (N=5). We validated this binary classification
model using 5-fold cross-validation. During this validation, the range of AUC
was (0.84–0.95) & the average AUC was 0.90. (Figure 2).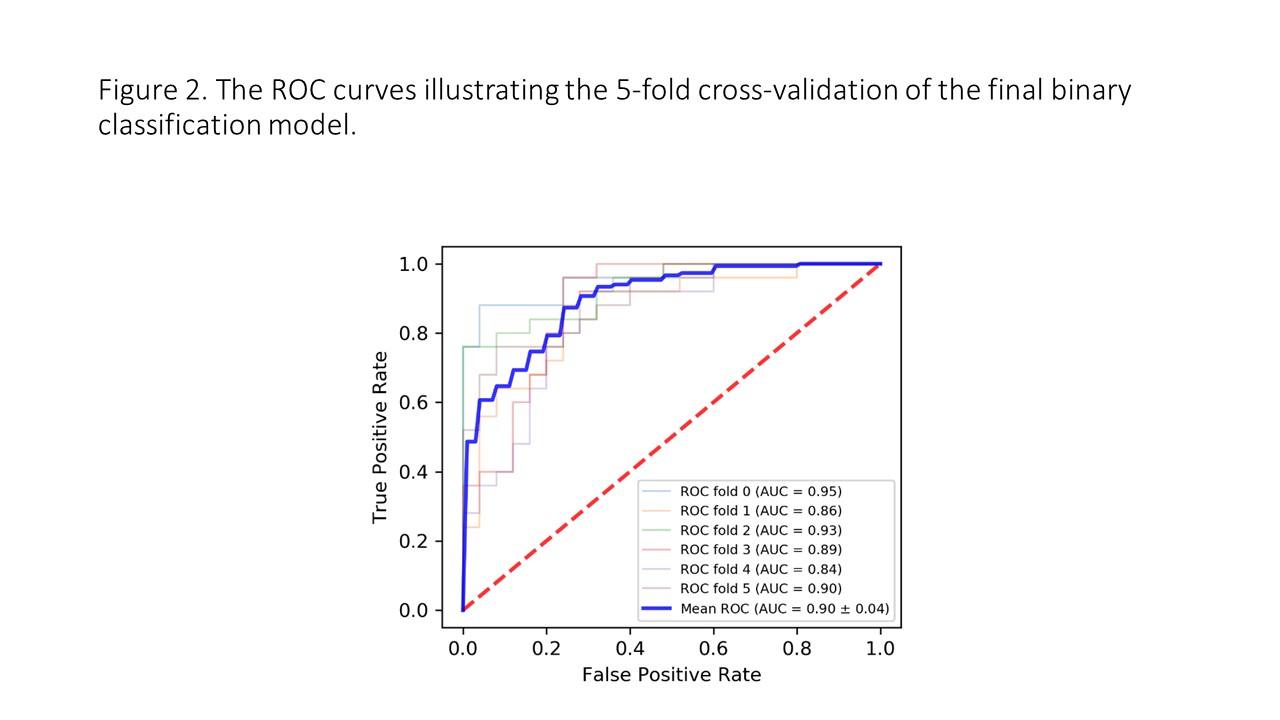
Conclusion
We successfully using imaging radiomic
features to construct an accurate model (AUC=
0.90) to discriminate ORN and
normal mandibular bone in head and neck cancer patients. Future studies are
needed to validate this model in prospective studies to early detect ORN in
head and neck cancer patients after radiation treatment.