Convolutional recurrent neural networks for future anatomy prediction
Denis Page,
United Kingdom
PO-1478
Abstract
Convolutional recurrent neural networks for future anatomy prediction
Authors: Denis Page1, Alan McWilliam1, Robert Chuter2, Andrew Green1
1University of Manchester, Division of Cancer Sciences, Manchester, United Kingdom; 2The Christie NHS Foundation Trust, Medical Physics and Engineering, Manchester, United Kingdom
Show Affiliations
Hide Affiliations
Purpose or Objective
Highly
conformal dose distributions allow for increased dose to target volumes without
a significant increase in dose to nearby organs at risk (OARs). Such dose
distributions can become unsafe to deliver due to changes in patient anatomy,
such as tumour shrinkage or weight loss. This mandates the implementation of
adaptive radiotherapy (ART), which can be resource intensive and time
consuming. In this work, we investigate the feasibility of using a
convolutional recurrent neural network (CRNN) to predict when replanning will
be necessary, allowing for efficient, ahead-of-time resource allocation.
Material and Methods
A time dependent CRNN was
developed capable of predicting the future anatomy of head and neck (HN) cancer
patients undergoing radiotherapy and the accuracy of predictions at different time
points in the future was investigated.
Series of 3D CBCTs obtained over
a course of treatment, which varied between 5 and 31 in length, from 266 HN
patients were used in this work, a total of 2849 images. For each series, the
scans were rigidly registered on the target region. The data was randomly split
into 70%, 20% and 10% groups for training, validation and testing respectively.
An input series lengths of 5, the number obtained in one week, along with the
date and time at which the scans were obtained, were presented to the network
and predictions of the next 10 scans were produced.
The accuracy of predictions was evaluated using
mean squared error (MSE) to compare predicted scans to ground truths.
Results
The
average MSE between the network predictions (Fig. 1C) and ground truths (Fig. 1B)
in a test set of 27 patients were 2.79x103 for predictions of
anatomy made 0-24 hours after the final input scan, 3.85x103 for
48-72 hours after, 6.54x103 for
144-168 hours after and 1.36x104 for 312-336 hours after. However, only 11 of
the 27 series in the test set contained 15 or more scans. The 144-168 hours and
312-336 hours results are averages of 22 and 11 prediction accuracies
respectively.
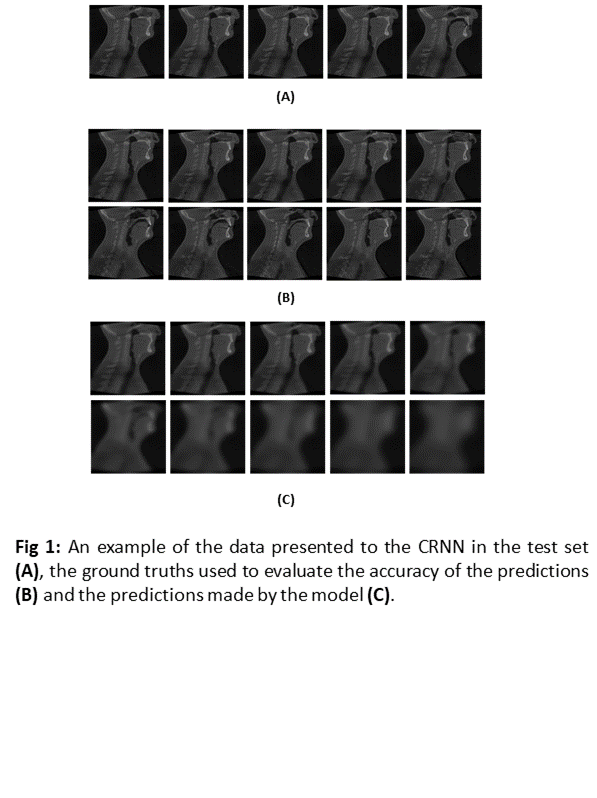
Conclusion
We
produced a CRNN architecture for predicting changes in head and neck patient
anatomy given one week of patient scans. Predictions of anatomy within 72 hours
of the final input image exhibit the modelling capabilities of the network. Further
work will focus on improving the model accuracy to provide useful anatomical
predictions for one week in the future and beyond, allowing for its use as a
decisions making tool, improving resource allocation.