Machine learning to predict locoregional relapse in pT1-2pN0-1 breast cancer following mastectomy
PO-1190
Abstract
Machine learning to predict locoregional relapse in pT1-2pN0-1 breast cancer following mastectomy
Authors: Stefania Volpe1, Federica Bellerba2, Mattia Zaffaroni1, Matteo Pepa1, Lars Johannes Isaksson1, Giorgia Maimone3, Bianca Menzani3, Ilaria Monaco3, Patrick Maisonneuve4, Ida Rosalia Scognamiglio1, Samantha Dicuonzo1, Maria Alessia Zerella1, Damaris Patricia Rojas1, Giulia Marvaso1, Cristiana Fodor1, Sara Gandini2, Elena De Momi3, Paolo Veronesi5, Giovanni Corso5, Viviana Enrica Galimberti5, Maria Cristina Leonardi1, Barbara Alicja Jereczek-Fossa1
1Istituto Europeo di Oncologia IRCCS, Radiation Oncology, Milan, Italy; 2Istituto Europeo di Oncologia IRCCS, Experimental Oncology, Milan, Italy; 3Politecnico di Milano, Electronics, Information and Bioengineering, Milan, Italy; 4Istituto Europeo di Oncologia IRCCS, Epidemiology and Biostatistics, Milan, Italy; 5Istituto Europeo di Oncologia IRCCS, Breast Surgery, Milan, Italy
Show Affiliations
Hide Affiliations
Purpose or Objective
While post-mastectomy radiotherapy is a mainstay
for the treatment of locally-advanced breast cancer patients, indications for early stages (namely, pT1-2 pN0-1) are less defined, and a clear understanding
of predictive factors of locoregional relapse (LRR) is warranted to better
establish clinical indications. This study explores the potentials of machine learning
(ML)-based algorithms in this clinical setting.
Material and Methods
A total of 2632 patients, treated at the
European Institute of Oncology IRCCS, Milan, Italy between 1998 and 2006, who
underwent mastectomy without subsequent radiotherapy was considered for the
analysis. Three ML- and
statistics-based regression models were trained to predict LRR and to estimate
the hazard ratios for all the predictor variables. For ML models the
importance of the clinical features on the outcome was estimated by permuting
out-of-bag (OOB) cases. The concordance index (c-index) was used to
compare the performances.
Results
A total of 1823 patients with no missing clinical
values was selected for the analysis and randomly split into training and validation
set (1367 and 456 patients, respectively, representing 75% and 25% of
the whole included population). The performance of the Cox’s proportional
hazard (CPH) model in the test set was 0.71, while the c-index of Random
Survival Forest (SRF) was 0.65 and the one of Survival Support Vector
Machine (SSVM) reached 0.67. Considering the validation set, the performance of
the CPH was comparable to those of SRF and SSVM, achieving c-indexes of 0.65,
0.65, and 0.67 in the validation test, respectively. Overall, the performance of
the Cox’s proportional hazard (CPH) model was comparable to those of Random
Survival Forest (SRF) and Survival Support Vector Machine (SSVM), achieving
c-indexes of 0.65, 0.65, and 0.67 in the validation test, respectively.
The most
significant contributions to the CPH model are shown in Figure 1A. The SRF confirmed the statistically significant
contribution of elevated Ki-67 (>20%), the primary tumor staging at surgery
(pT), and the execution of any systemic treatment. The combination of risk
factors and molecular subtypes also provided a significant contribution to the
model, together with young age (<35 years). A graphical representation of
variable importance is SRF is reported in Figure 1B.
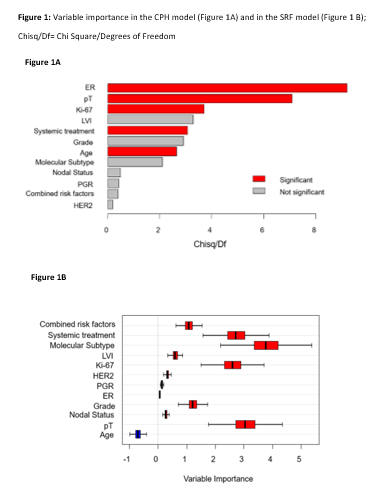
Conclusion
The prediction accuracy between CPH and ML
algorithms in terms of C-index was comparable in both the test and validation
sets. Overall, results of CPH were largely confirmed by those of SRF, with
clinically-meaningful estimates of variables contribution for the prediction of
LRR. The quantitative assessment of the importance of individual parameters in SSVM is
more challenging. In perspective, external validation would be beneficial to
confirm our results.