A novel method for evaluating CBCT-based synthetic CTs
Chelsea Sargeant,
United Kingdom
PD-0071
Abstract
A novel method for evaluating CBCT-based synthetic CTs
Authors: Chelsea Sargeant1, Andrew Green1, Robert Chuter1,2, Alan McWilliam1
1The University of Manchester, Division of Cancer Sciences – Faculty of Biology, Medicine and Health, Manchester, United Kingdom; 2The Christie NHS Foundation Trust, Christie Medical Physics and Engineering, Manchester, United Kingdom
Show Affiliations
Hide Affiliations
Purpose or Objective
Artificial intelligence (AI) models are increasingly used to generate synthetic CTs (sCTs) from on-treatment CBCTs and hold potential for adaptive radiotherapy. However, lack of a ground truth image hinders the evaluation of sCTs. Commonly, the planning CT (pCT) is deformable registered to the CBCT anatomy to create a deformed CT (dCT) for comparison, but this suffers from inherent uncertainties due to the registration. We propose a novel method to evaluate sCTs using the differences between scans to isolate model and registration accuracy and determine whether sCT is superior to dCT.
Material and Methods
For 10 prostate patients, the pCT and all on-treatment CBCTs (range: 6-12 per patient, total 92 images) were collected. ADMIRE v3.29.1 (Elekta AB) was used to generate the dCTs and sCTs for each CBCT; a cycle Generative Adversarial Network (cycleGAN) was used for sCT generation, while a b-spline based registration engine was used to produce dCT.
For each time point, the pCT, CBCT, dCT and sCT were rigidly registered and the differences determined. These differences are shown in Fig 1 and are assumed to be derived from the inherent differences between the scans: anatomical differences, image quality, model error and registration error. Each image was masked according to the smallest field of view across modalities
The mean pixel value for each difference was calculated for each time point per patient and compared using a paired intra-patient Mann-Whitney U test. We test for the alternative hypothesis to determine if mean difference in the dCT arm of the comparison is significantly greater than the sCT arm (greater value corresponds to worse performance).
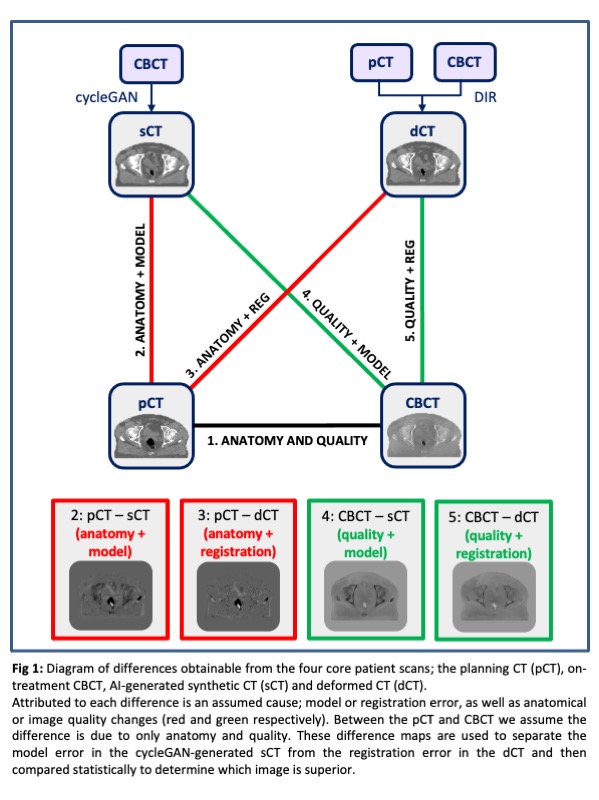
Results
In 80% of cases, the sCT outperforms the dCT as indicated by a significantly smaller average pixel value in the model arm of comparisons than the registration arm (Fig 2A).
Compared to all other patients, Patients 2 and 6 suffer from severe artefacts across each of the CBCTs. The cycleGAN does not adequately suppress these artefacts, impacting the sCT while the dCT remains unaffected (Fig 2B). While the cycleGAN model was trained with a large number of CT-CBCT prostate pairs, the model appears not to generalise to artefacts in the CBCT, implying a scarcity of artefacts in the training data.
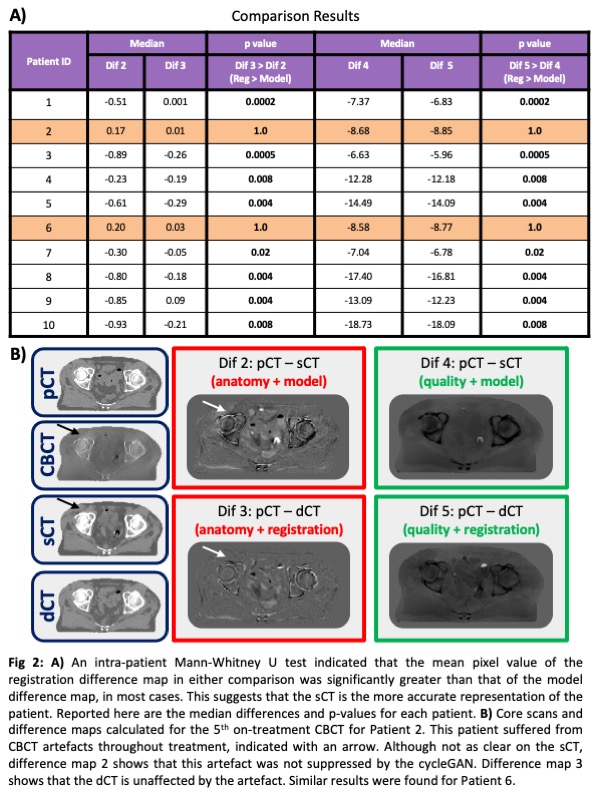
Conclusion
We present a novel method to evaluate sCTs that considers inherent uncertainties in generating the test data. cycleGAN-generated sCTs outperform the equivalent dCT in terms of image similarity, but the model fails to suppress the impact of severe CBCT artefacts, and the dCT is a more suitable choice for these patients. There is a need for further study into the behaviour of image synthesis models when artefacts are present. This analysis should be repeated with a larger, curated dataset, and a comparison against existing metrics is required. Overall, our method successfully identifies cases where a sCT is an improvement on the conventional dCT.