Predicting early mortality using muscle characteristics for patients with lung cancer
MO-0391
Abstract
Predicting early mortality using muscle characteristics for patients with lung cancer
Authors: Alan McWilliam1, Donal McSweeney2, Kathryn Banfill1, Marcel van Herk1, Corinne Faivre-Finn3, Andrew Green1
1University of Manchester, Division of Cancer Science, Manchester, United Kingdom; 2University of Manchester, Division of Cancer Science, Mancheter, United Kingdom; 3Univesity of Manchester, Division of Cancer Science, Manchester, United Kingdom
Show Affiliations
Hide Affiliations
Purpose or Objective
There is a need for quantitative biomarkers to guide
treatment decisions in patients with lung cancer treated with radiotherapy.
Decisions on treatment intent consider variables, such as age, stage and
performance status, but predicting early mortality is challenging. 90-day
mortality is used in surgical decision making, but no models have been developed
for radiotherapy. In this work, we investigated
muscle characteristics as an image-based biomarker to predict early mortality.
Material and Methods
586
patients with NSCLC lung cancer treated with 55Gy in 20 fractions were
identified from a retrospective database. The muscle compartment at T12 was
segmented on planning CT using an in-house AI model. Muscle area and density
were extracted for all patients. Early mortality was defined as death within 90-days
of start of treatment. A logistic regression model predicting early mortality
was built including known prognostic variables and variables that impact muscle
quality; age, sex, performance status, T-stage, and N-stage. Models with muscle
area, muscle density and performance status were individually built. 5-fold
cross-validation was used to assess model accuracy and Akaike Information Criteria
(AIC) ranked model performance.
Results
39 segmentations failed leaving 547 patients for analysis.
Patient characteristics are included in table 1. Median muscle area of 40.4cm3
(11-110cm3) and median density 12.7HU (-7.4-34.2HU) were found,
indicating poor muscle health across the population. 64 (11.7%) patients died
within 90-days of treatment, higher than reported in surgical series. These patients
had significantly lower muscle density (Mann-Whitney, p=0.03) but not muscle area (Mann-Whitney, p=0.28). Individual
logistic regression models showed significance of muscle density (p=0.02) and
muscle area (p=0.003), reduction in muscle was associated with early mortality.
Male sex was significant in both models, accounting for age, T-stage, N-stage
(all non-significant), table 2. Male sex
showed a significant difference in muscle characteristics (Mann-Whitney,
density p=0.04 and area p=0.004) indicating this effect is driven by gender
(figure 1a).
5-fold cross-validation showed model accuracy
with muscle density of 0.87 and for the model with muscle area an accuracy of
0.88. The AIC for the logistic regression including muscle area was significantly
improved compared to the model including density. Finally, the model with
performance status showed no significant association with ninety-day mortality.
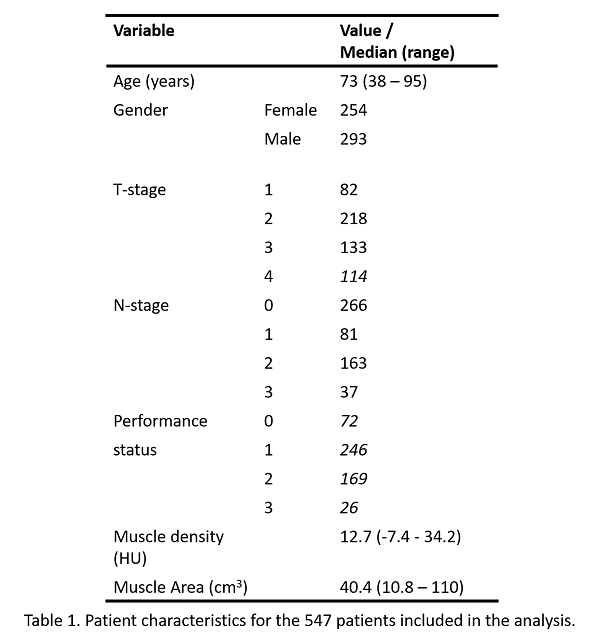
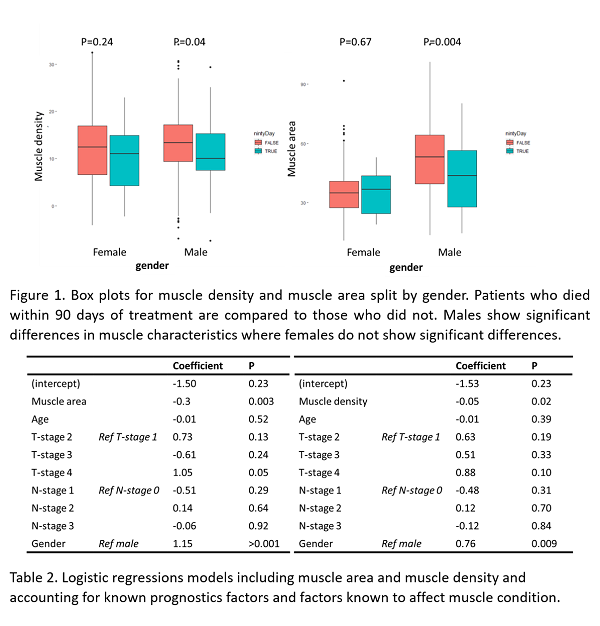
Conclusion
We have shown that muscle characteristics, collected from routine imaging, are
a potential tool for predicting 90-day mortality for patients with lung cancer
treated with radiotherapy. Model performance showed good accuracy with muscle
area or density, with males showing greater impact of poor muscle condition on
90-day mortality. Muscle characteristics showed superior predictive performance
over performance status. Further internal and external validation will be
performed.