Comparative of different dose prediction and robust mimicking strategies for automatic IMPT planning
Elena Borderias-Villarroel,
Belgium
OC-0451
Abstract
Comparative of different dose prediction and robust mimicking strategies for automatic IMPT planning
Authors: Elena Borderias-Villarroel1, Margerie Huet-Dastarac1, Ana María Barragán-Montero1, Mats Holmstrom2, Xavier Geets1,3, Edmond Sterpin4,5
1UCLouvain, MIRO, Brussels, Belgium; 2RaySearch Laboratories, Machine Learning, Stockholm, Sweden; 3Cliniques Universitaires Saint Luc, Radiation therapy , Brussels, Belgium; 4UCLovain, MIRO, Brussels, Belgium; 5KULeuven, Laboratory of Experimental Radiotherapy, Department of Oncology, Leuven, Belgium
Show Affiliations
Hide Affiliations
Purpose or Objective
Automatic planning based on dose prediction with deep learning followed by dose mimicking is becoming popular. The term dose mimicking refers to the process of finding, through conventional optimization algorithms, the machine parameters that deliver a reference three-dimensional dose distribution. In proton therapy, dose mimicking is even more challenging, because the optimization algorithm needs to both accurately reproduce the dose distribution and the associated robustness against uncertainties. This study compares the performance of three different automatic planning workflows: (1) our in-house prediction and mimicking solutions (IH = IHpred-IHmim), (2) the ones from the commercial treatment planning system RayStation10B (RaySearch Laboratories, Sweden) (RS = RSpred-RSmim) and (3) a hybrid combination of both (HYB = IHpred-RSmim).
Material and Methods
Our database consisted of 60 bilateral head and neck cancer (HNC) patients planned with robust intensity-modulated proton therapy (IMPT). Prediction models (RSpred and IHpred) used the same training (50), validation (5), and test (5) data sets. Both prediction models were based on U-Net architecture. Our IHmim used isodose contours generated from the predicted doses and minimum and maximum optimization objectives to replicate the predicted dose distribution in the planning-CT. RayStation mimicking strategy, RSmim, was an optimization method mixing voxel-wise optimization and composite loss function with weighted dose-volume objectives. For the 5 test patients, nominal and worst-case values for target coverage (D98% > 95%Prescription) and OAR clinical goals were reported from robustness evaluations of the automatically generated plans (IH, RS, HYB) and compared to the values from the clinical plan.
Results
Figure 1. shows the target coverage differences with respect to reference in two clinical target volumes (CTVs) with different prescriptions. For the three RS, HYB and IH automatic planning strategies, target coverage in low-dose and high-dose CTVs was replicated within [-0.65, 0.95] Gy and [-1.78, 0.88] Gy difference with respect to planning for nominal and worst-case, respectively. Higher differences were observed for the OARs, sparing of the spinal canal and the parotid differed between RS and IH while HYB found a compromise with median values closer to the clinical plan (Figure 2). Superior robustness level (i.e higher worst-case values), in low-dose target coverage was observed for HYB with respect to RS and IH.
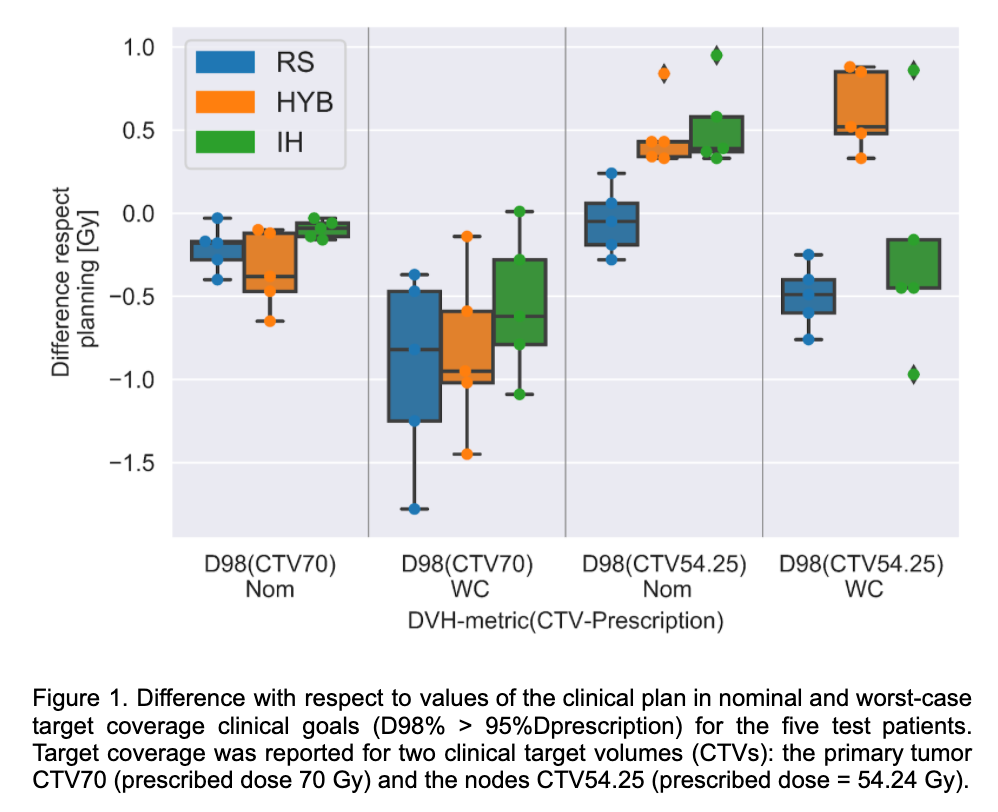
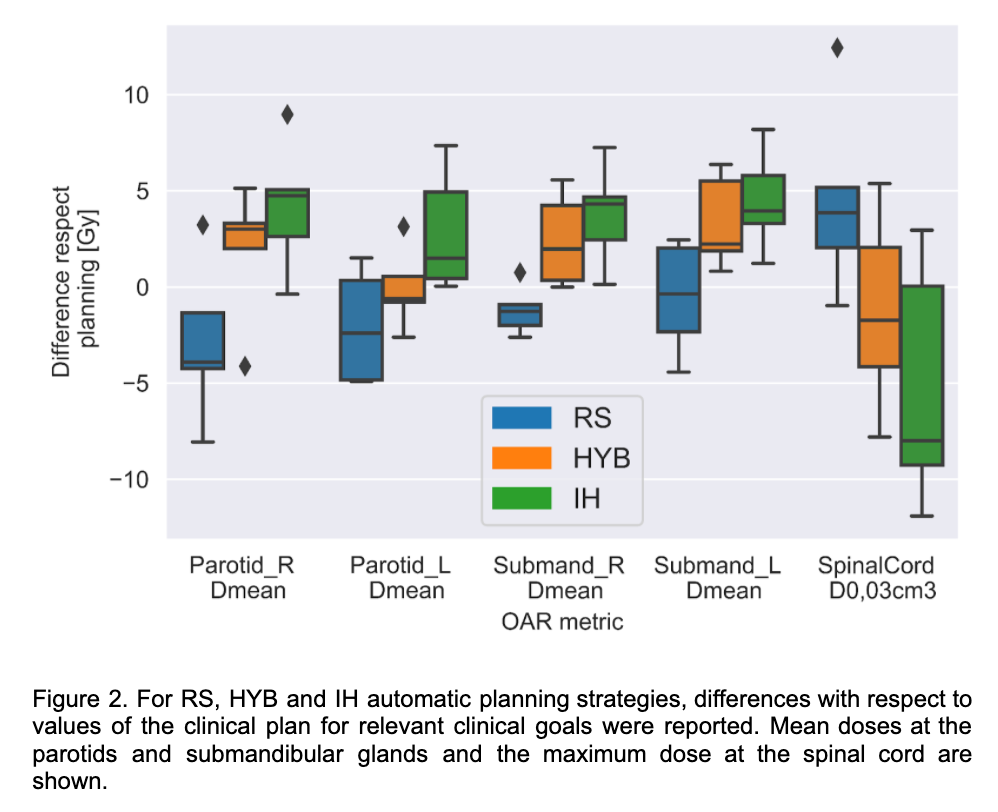
Conclusion
RSM, HYB and IH automatically generated plans obtained satisfactory mimicked nominal doses with results acceptably similar to the clinical reference dose. However, this study showed the importance of analysing the key step of robust dose mimicking in automatic planning to assess OAR toxicity and robustness of the chosen strategy.