Recovery of dose profiles of small photon fields in magnetic field using artificial neural networks
OC-0119
Abstract
Recovery of dose profiles of small photon fields in magnetic field using artificial neural networks
Authors: Hui Khee Looe1, Isabel Blum1, Ann-Britt Schönfeld2, Tuba Tekin1, Björn Delfs2, Björn Poppe2
1Carl von Ossietzky University, University Clinic for Medical Radiation Physics, Oldenburg, Germany; 2Carl von Ossietzky University , University Clinic for Medical Radiation Physics, Oldenburg, Germany
Show Affiliations
Hide Affiliations
Purpose or Objective
The feasibility to apply deep learning for the recovery of unperturbed dose profiles from measured signals subject to detector perturbation in the presence of a magnetic field has been explored. Artificial neural networks (NN) are trained using high-quality data generated based on an established mathematical convolution model, hence eliminating the necessity of time- and labor-intensive acquisition of training data.
Material and Methods
The investigations were performed using four detectors: two air-filled ionization chambers (PTW Semiflex 3D 31021 und PinPoint 3D 31022 chambers) and two diode-type detectors (PTW microDiamond 60019 and microSilicon 60023). Using an efficient simulation technique introduced previously (Looe et al 2018 Phys Med Biol. 63(7):075013.), 2D dose deposition kernels and detector lateral fluence response functions of all four detectors have been obtained for B = 0, 0.35 and 1.5 T. By applying these convolution kernels, training data consisting of pairs of 2D dose profiles D(x,y) and measured signal profiles M(x,y) were generated for a set of 48 fluence profiles that cover different field sizes and penumbra widths. Three-layer feed-forward NN were trained individually for each detector and magnetic field. The resulted NN were tested with a distinct set of fluence profile, and additionally, with profiles simulated using realistic Monte Carlo phase space files (PSF) of clinical linear accelerator.
Results
Due to the influence of the Lorentz force on the electron trajectories, the simulated dose deposition kernels and detector lateral fluence response functions are magnetic field dependent. The NN mean square errors (MSE) approached values of 1e-6 or better during the training and validation. All models also attained correlation coefficients R near 1 indicating an excellent agreement between expected D(x,y) and predicted Dpred(x,y). The gamma indices (1mm/1% global) of all evaluated points in the test profiles passed (< 1). Fig. 1 shows exemplarily the results of the test profiles simulated using the PSF of 10 mm x 10 mm field size in a 1.5 T magnetic field.
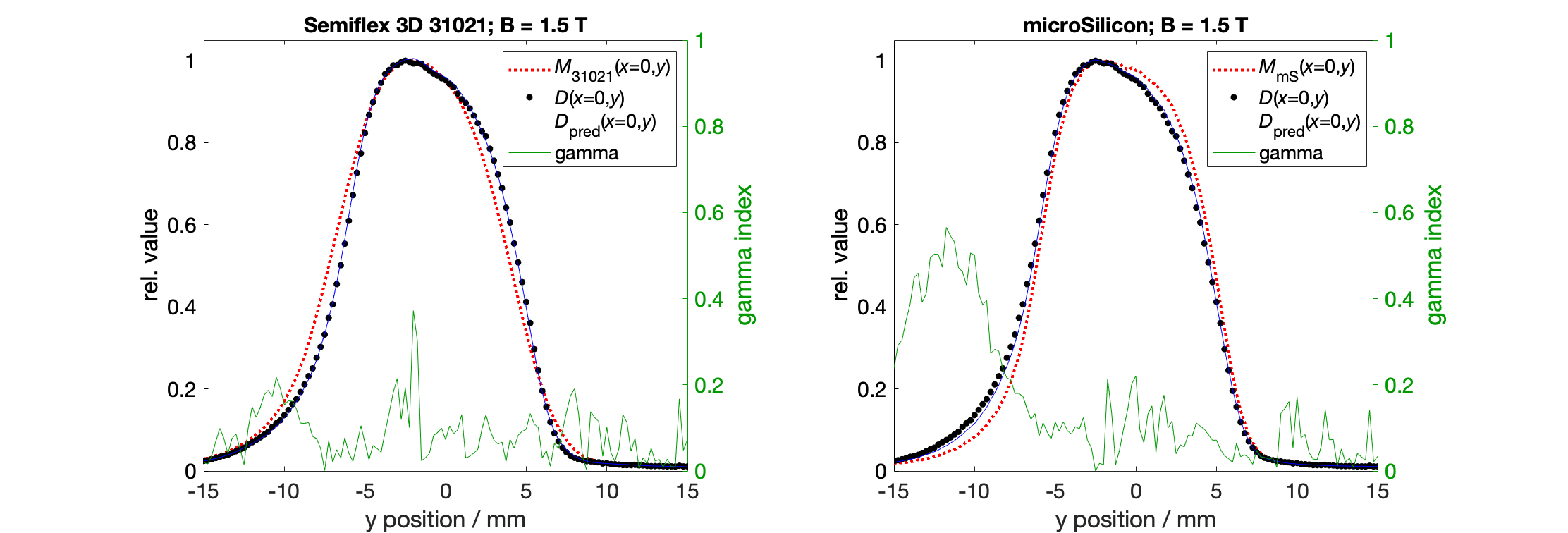
Fig. 1. Comparisons of the expected D(x,y) and NN predicted Dpred(x,y) from the measurement using Semiflex 3D 31021 and microSilicon in a 1.5 T magnetic field simulated using the PSF of 10 mm x 10 mm field size
Conclusion
A model-based measurement-less training approach has been successfully implemented and tested to generate NN that can recover lateral dose profiles from distorted measurements in a magnetic field. Furthermore, two different verification approaches have been proposed that allow users to warrant the mathematical consistencies of the predicted results within the framework of the convolution model. Besides offering a clinical feasible correction strategy not existed so far for relative dosimetry in a magnetic field, this work can also help to raise awareness and to improve understanding on the distortion of detector’s signal profiles by a magnetic field.