A cohort of 56 patients was available for this study. Patients were
treated for LACC according to the EMBRACE2 protocol. An open MR-scanner (0.35T)
was used to acquire the images, with in-plane resolution 256x256 (1.17 mm), and
5 mm slice thickness.
For each patient two BT fraction treatment plans, including MRI and reconstructed applicators were exported. The
contours of the applicator, which are usually not available in the TPS
(Oncentra Brachy, Elekta), were generated with an Elekta Applicator Slicer
research plugin and treated as ground-truth masks.
Automatic registration of the image pairs was performed with five
different registration algorithms written in Python. Tab. 1 provides an
overview of the different registration configurations.
As ground-truth masks would not be available in clinical routine, a NN
(UNET, implemented in PyTorch) was trained to predict the applicator structure
in previously unseen MR-images. Training was performed using 5-fold cross
validation and a random train-test split.
The best performing registration algorithm was re-run with the output of
the NN, to compare the effect of ground-truth vs predicted masks.
Registration results were evaluated on the test set, using the root mean
squared error of the applicator dwell positions (RMSE). Performance of the NN
was measured using the DICE-coefficient.
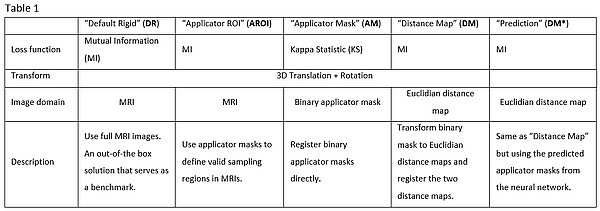