Auto-contouring of cardiac avoidance region for cardiac sparing lung radiotherapy
Tom Marchant,
United Kingdom
PO-1653
Abstract
Auto-contouring of cardiac avoidance region for cardiac sparing lung radiotherapy
Authors: Tom Marchant1,2, Gareth Price2, Alan McWilliam2, Edward Henderson2, Donal McSweeney2, Kathryn Banfill2,3, Jennifer King3, Claire Barker3, Corinne Faivre-Finn2,3
1The Christie NHS Foundation Trust, Christie Medical Physics & Engineering, Manchester, United Kingdom; 2The University of Manchester, Division of Cancer Sciences, Manchester, United Kingdom; 3The Christie NHS Foundation Trust, ., Manchester, United Kingdom
Show Affiliations
Hide Affiliations
Purpose or Objective
Heart dose during lung radiotherapy is increasingly recognised to have a significant effect on patient outcome. Several studies have identified an area at the base of the heart where dose is strongly correlated with overall survival. To introduce a dose limit for this cardiac avoidance area (CAA) it first needs to be contoured for treatment optimisation. An auto-contouring solution for this region will reduce the time spent contouring by clinicians, improve contouring consistency, and make implementation of heart sparing radiotherapy feasible. In this abstract we report the development and validation of a deep-learning based auto-contouring method for the proposed CAA.
Material and Methods
The CAA chosen at our institution includes the right atrium, aortic valve root, and proximal portions of the left and right coronary arteries. An existing fast, efficient 3D Convolutional Neural Network [DOI: 10.1016/j.media.2022.102616] was adapted for cardiac substructures. The model is designed for training with a small dataset, here 42 4DCT-AVG scans of patients with lung cancer with manual contours for CAA and associated subregions. When trained, contours are produced using CPU only, facilitating easy deployment of the model without specialist hardware. An initial step identifies the heart location in the CT and crops the image around it [DOI: 10.48550/arXiv.2209.06042]. Contouring runs on the cropped image to infer masks representing each cardiac substructure, before these are combined into the final CAA contour. Auto-contour quality was assessed by geometric comparison to manual contours for 10 patients not used for training. Further, auto-contouring robustness was evaluated using 198 CT scans. Forty-five randomly selected cases were rated by an expert clinician using a 1-5 scale (1=no output/crash, 2=poor/needs contouring from scratch, 3=OK/significant changes needed, 4=Good/Minor changes needed, 5=Very good/no changes needed).
Results
Figure 1 shows manual vs automatic CAA contours for the 10 validation patients. Mean Dice similarity coefficient for the whole CAA was 0.77±0.09. The clinician observer rated auto-contours as “Good” in 35/45 cases, with the remaining 10 cases rated as “OK”. The auto-contouring software ran successfully for all 198 CT scans used for robustness testing. Each auto-contour was produced in ~1s on an Intel Xeon 6134 CPU, although the full processing pathway took 30-60s (includes data in/output, cropping and post-processing).
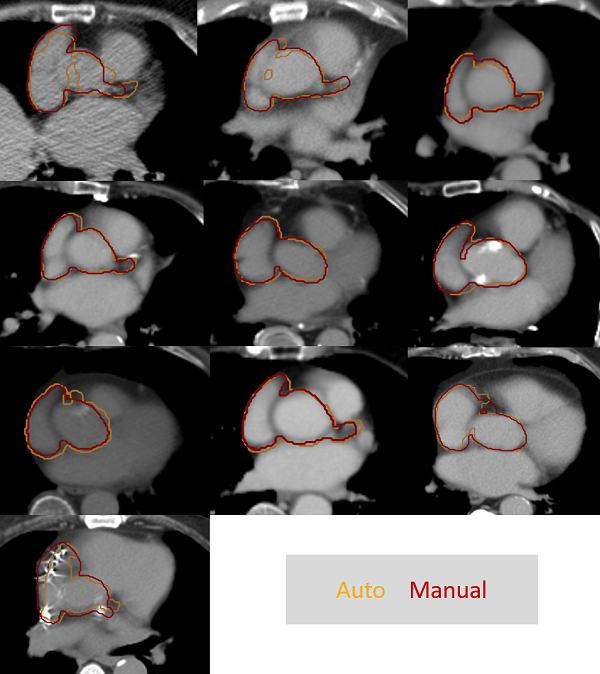
Figure 1: Comparison of manual vs automatic CAA contours.
Conclusion
A fast and robust auto-contouring solution for the CAA was developed and validated. Auto-contour quality was rated as “Good” in most cases, requiring minimal editing, allowing fast CAA contouring. Accurate and consistent CAA contouring will support the introduction of heart-sparing RT as part of the RAPID-RT study. This study will assess the impact of cardiac sparing RT on survival in patients having radical radiotherapy for lung cancer [DOI: 10.1016/j.clon.2021.12.017].