A 3D transfer learning approach for identifying multiple simultaneous errors during radiotherapy
PO-1650
Abstract
A 3D transfer learning approach for identifying multiple simultaneous errors during radiotherapy
Authors: Cecile Wolfs1, Kars van den Berg2, Frank Verhaegen1
1GROW School for Oncology - Maastricht University Medical Center, Radiation Oncology (Maastro), Maastricht, The Netherlands; 2Eindhoven University of Technology, Department of Biomedical Engineering, Medical Image Analysis group, Eindhoven, The Netherlands
Show Affiliations
Hide Affiliations
Purpose or Objective
Advancements in radiotherapy (RT) led to more complex and precise treatment, causing in vivo verification to become increasingly important. Artificial intelligence (AI) algorithms, such as deep learning (DL), present an opportunity for automatic processing of the large amounts of multi-dimensional data associated with in vivo verification. DL models such as convolutional neural networks (CNNs), can take full dose comparison images as input and have shown promising results for identification of single errors during treatment (Wolfs et al. (2020) Radiother Oncol). Clinically, complex scenarios should be considered, with the risk of multiple anatomical and/or mechanical errors occurring simultaneously during treatment. The purpose of this study was to evaluate the capability of CNN-based error identification in this more complex scenario.
Material and Methods
For 40 lung cancer patients, clinically realistic ranges of possible combinations of various treatment errors within the treatment plans and/or CT images were simulated. The modified CTs and treatment plans were used to predict a total of 2560 3D dose distributions, which were compared to the dose distributions without errors using (3%, 3 mm) gamma analysis and relative dose differences. 3D CNNs capable of multilabel classification (i.e., an input sample can be assigned to more than one class) were trained to identify treatment errors at two classification levels, using the dose comparison volumes as input: Level 1 (main error type, e.g. anatomical change, mechanical error) and Level 2 (error subtype, e.g. tumor regression, patient rotation). For training the CNNs, a transfer learning approach was employed, as illustrated in Figure 1. An ensemble model was also evaluated, which consisted of three separate CNNs that each took a subset of the dose comparison volume as input, based on the body, lung and PTV contours. Model performance was evaluated by calculating the sample F1-score for training and validation sets.
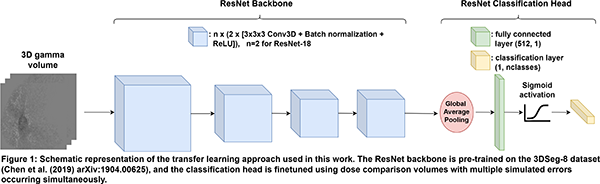
Results
Table 1 shows that the baseline model already has high F1-scores for Level 1 classification, but that performance for Level 2 is lower, and overfitting becomes more apparent. Using relative dose difference volumes as input improves performance for Level 2 classification, whereas using an ensemble model additionally reduces overfitting.
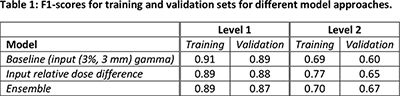
Conclusion
This study shows that it is possible to identify multiple errors occurring simultaneously in 3D dose verification data. While classification of the main error type shows high performance, classification of the error subtype improves by using relative dose difference volumes and ensemble models that take dose comparison volumes in different anatomical structures into account.