Novel dataset validation of deep learning models for autocontouring of head and neck, and prostate
Daniel Sandys,
United Kingdom
PO-1647
Abstract
Novel dataset validation of deep learning models for autocontouring of head and neck, and prostate
Authors: Daniel Sandys1, Naomi Fersht2, Anna Thompson2, Reena Davda2, Sabina Khan3, Melissa Bristow4, Peter Hessey4, Anton Schwaighofer4
1University College London Hospitals NHS Foundation Trust, Radiotherapy Physics, London, United Kingdom; 2University College London Hospitals NHS Foundation Trust, Oncology, London, United Kingdom; 3University College London Hospitals NHS Foundation Trust, Radiotherapy and Proton Beam Therapy, London, United Kingdom; 4Microsoft Research, Health Futures, Cambridge, United Kingdom
Show Affiliations
Hide Affiliations
Purpose or Objective
Manual contouring in radiotherapy is a major time and cost demand. Additionally, interobserver variability (IOV) is a major contributor to treatment variance in radiotherapy. Automated contouring (autocontouring) has the potential to provide consistent contours with substantially reduced demand on resources. Previous work has shown it is possible to train deep learning models to generate autocontours which agree with manual clinician contours within the range of clinician IOV.
This work assesses the quality of two previously trained autocontouring models on a novel retrospective patient cohort, from a clinical centre which did not provide training data.
Material and Methods
Models (InnerEye, Microsoft Research) were previously trained on head and neck (H&N), and prostate patient cohorts contoured according to EORTC and TROG guidelines. This validation work assessed these models against a retrospective cohort of H&N (n=20) and prostate (n=20) patients treated between 2015-2018 and 2019-2020 respectively.
The H&N cohort were treated with EBRT for 65Gy in 30#, or 70Gy in 35#. The prostate cohort were treated with EBRT for 60Gy in 20# to the prostate and seminal vesicles (excluding post-operative or pelvic lemph node radiotherapy).
Manual clinician contours were generated according to local contouring guidelines and collected retrospectively. Manual and autocontours were compared using Dice similarity coefficient and Hausdorff distance. Manual contours from the local clinical centre acted as ground truth for comparison.
Results
Autocontours were generated for each patient (Fig. 1). Here a subset of results is presented (Fig. 2), showing agreement with local clinicians is consistent with previously published results for these models. Statistics which deviate from previously published values by are highlighted. These aggregate statistics indicate results which are broadly consistent with those indistinguishable from IOV.
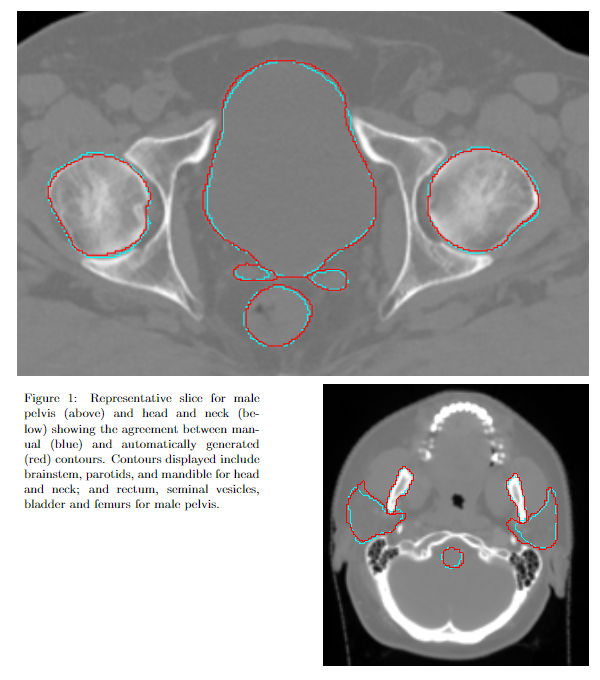
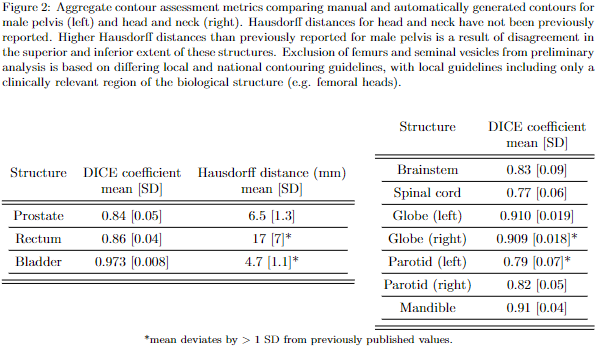
Conclusion
This work demonstrates that previously trained autocontouring models show a consistent level of accuracy when applied to a previously unseen dataset from a novel clinical centre. Hence, the potential has been demonstrated for these previously trained models to be clinically viable in the local centre. This would reduce the resource demand associated with contouring and reduce variability in patient treatment which results from manual contouring.
Limitations include available computational resources forcing a patch-based approach to classification, which may degrade the quality of output contours. Discrepancies between manual and autocontours tended to occur in the superior and inferior extent of tubular structures or at structure interfaces, as previously reported; or where local guidelines differ from national guidelines, which has caused preliminary exclusion of some structures from this analysis.
Further work will include a prospective assessment of time savings delivered by autocontouring.