MRI-based deep learning autocontouring: Evaluation and implementation for brain radiotherapy
Nouf Alzahrani,
United Kingdom
PO-1640
Abstract
MRI-based deep learning autocontouring: Evaluation and implementation for brain radiotherapy
Authors: Nouf Alzahrani1,2, Ann Henry3, Anna Clark4, Bashar Al-Qaisieh4, Louise Murray5, Michael Nix4
1Leeds Cancer Center and University of Leeds, Medical Physics and Clinical Engineering, Leeds, United Kingdom; 2Diagnostic Radiology Department, Faculty of Applied Medical Sciences, King Abdulaziz University,KSA, Diagnostic Radiology , Jeddah, Saudi Arabia; 3Leeds Cancer Centre, St. James’s University Hospital and University of Leeds, Radiotherapy, Leeds, United Kingdom; 4Leeds Cancer Centre, St. James’s University Hospital, Medical Physics and Clinical Engineering, Leeds, United Kingdom; 5Leeds Cancer Centre, St. James’s University Hospital and University of Leeds, Radiotherpay, Leeds, United Kingdom
Show Affiliations
Hide Affiliations
Purpose or Objective
There is great demand for auto-segmentation methods, to standardize and enhance the contour quality, and improve efficiency by automating clinical workflows. There are several recent commercially available deep learning auto-segmentation models to delineate OARs for head and neck but not for brain MRI, which is superior to CT for brain structure visualisation.
The main objective of this study is to build and evaluate an MRI autosegmentation model in RayStation for brain OARs. We also investigate the impact of contour editing prior to training on model performance. We assess geometric and dosimetric quality, to ascertain clinical usability.
Material and Methods
41 glioma patients were randomly selected for model training and validation. OARs for autosegmentation were brainstem, cochlea, orbits, lenses, optic chiasm, optic nerves, lacrimal glands, and pituitary gland. OARs were contoured according to a clinical consensus atlas.
MRI autosegmentation models were trained using a 3D U-net architecture (RayStation 11A - Raysearch). Three models were trained, using: i) Original clinical contours from planning CT, rigidly registered to T1-weighted gadolinium enhanced MRI (MRI unedited). ii) Original clinical contours edited on CT, to adhere to the local guidelines (CTe-MRI). iii) CT-edited clinical contours further edited based on the MRI anatomy (MRIe-MRI).
The performance of the three models was geometrically and dosimetrically, evaluated and compared to the gold standard (clinical contour edited on MRI) Dice similarity coefficient (DSC), and mean distance to agreement (MDA) were used for geometric evaluation. D1%, D5%, D50%, and maximum dose metrics were used for dosimetric evaluation. Models were compared by paired Student’s T-testing.
Results
The MRIe-MRI model successfully segmented more OARs, with higher DSC (10/13 OARs) and lower MDA, except for lacrimal glands, where CTe-MRI performed better. Paired T-test for DSC showed pituitary, orbits, optic nerves, and optic chiasm were significantly improved for MRIe-MRI vs. CTe-MRI. Also, it showed pituitary, orbits, optic nerves, and left lens was statistically different after comparing the MRIe-MRI model to MRI unedited model.
Where less than 6 cases were successfully segmented, the model segmenting more cases was preferred. MRIe-MRI model segmented more cochlea and lenses. CTe-MRI segmented more lacrimal glands.
There was no statistically significant dosimetric difference between the models and only weak correlation between geometric and dosimetric measures.
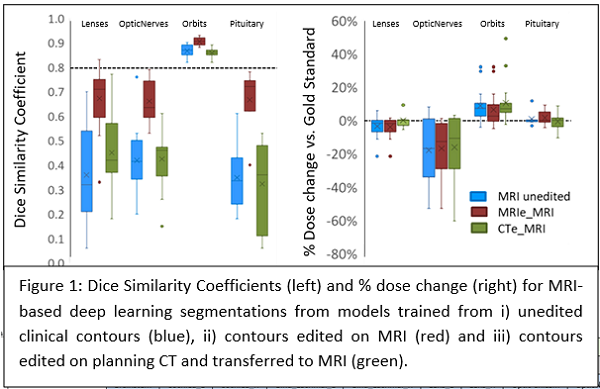
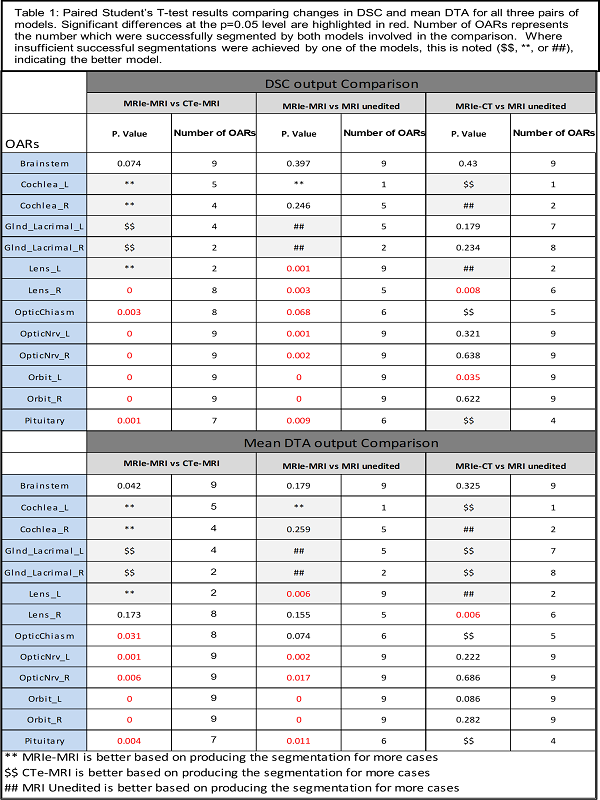
Conclusion
MRI deep learning auto segmentation of brain OARs can segment all OARs except the lacrimal glands, which are hard to visualise on MRI. Editing clinical contours on MRI before model training improves performance. Implementing an MRI deep learning auto-segmentation in the RT pathway will improve contour consistency and efficiency but requires careful editing of OARs on MRI.