deep learning-based automatic segmentation of rectal tumors in endoscopy images
Alana Thibodeau-Antonacci,
Canada
PO-1632
Abstract
deep learning-based automatic segmentation of rectal tumors in endoscopy images
Authors: Alana Thibodeau-Antonacci1,2, Luca Weishaupt3, Aurélie Garant4, Corey Miller5,6, Té Vuong7, Philippe Nicolaï2, Shirin Enger1,3
1McGill University, Medical Physics Unit, Montreal, Canada; 2Université de Bordeaux, Centre Lasers Intenses et Applications (CELIA), Bordeaux, France; 3Jewish General Hospital, Lady Davis Institute for Medical Research, Montreal, Canada; 4UT Southwestern Medical Center, Department of Radiation Oncology, Dallas, USA; 5McGill University, Department of Medicine, Montreal, Canada; 6Jewish General Hospital, Division of Gastroenterology, Montreal, Canada; 7Jewish General Hospital , Department of Oncology, Montreal, Canada
Show Affiliations
Hide Affiliations
Purpose or Objective
Radiotherapy is commonly used to treat rectal cancer. Accurate tumor delineation is essential to deliver precise radiation treatments. Endoscopy plays an important role in the identification of rectal lesions. However, this method is prone to errors as tumors are often difficult to detect. Previous deep learning methods to automatically segment malignancies in endoscopy images have used single expert annotations or majority voting to create ground-truth data, but this ignores the intrinsic inter-observer variability associated with this task. The goal of this study was to develop an unbiased deep learning-based segmentation model for rectal tumors in endoscopy images.
Material and Methods
Three annotators identified tumors and treatment change (i.e., radiation proctitis, ulcers and tumor bed scars) in 464 endoscopy images from 18 rectal cancer patients. In cases where the image quality was too low to confidently classify the tissues, the image was labeled as “poor quality”. The inter-observer variability was evaluated for whole image classification and on a contour level. A deep learning model was trained with all annotators’ labels simultaneously to perform pixel-wise classification. Grouped stratified splitting was used to divide the data into the training (375 images; 1125 labels), validation (29 images; 87 labels) and test (60 images; 180 labels) sets. A dice loss function was used to train a DeepLabV3 model with a pre-trained ResNet50 backbone. Automatic hyperparameter tuning was performed with Optuna. Images were normalized according to the COCO standard and resized to 256x256 pixels. Random transformations (horizontal and vertical flipping, rotation) were applied once per epoch to the training set with a 50% probability to increase the robustness of the model.
Results
Significant variability was observed in the manual annotations as shown in Figure 1. There was greater disagreement for treatment change. The deep learning model was able to segment each image in the test set in less than 0.05 seconds on CPU, while manual contours took on average 21 seconds per image. The model achieved an average dice score of 0.7526 and 0.6438 for tumor and treatment change, respectively. Figure 2a shows a case where the model accurately identified a tumor. In Figure 2b, the model segmented a tumor and treatment change even though majority voting (top left) identified these regions as “unclassified”. By considering all labels simultaneously, the model was able to reflect the fact that one annotator thought there was a tumor in the image and that another contoured treatment change. Figure 2c shows an example where the model failed.
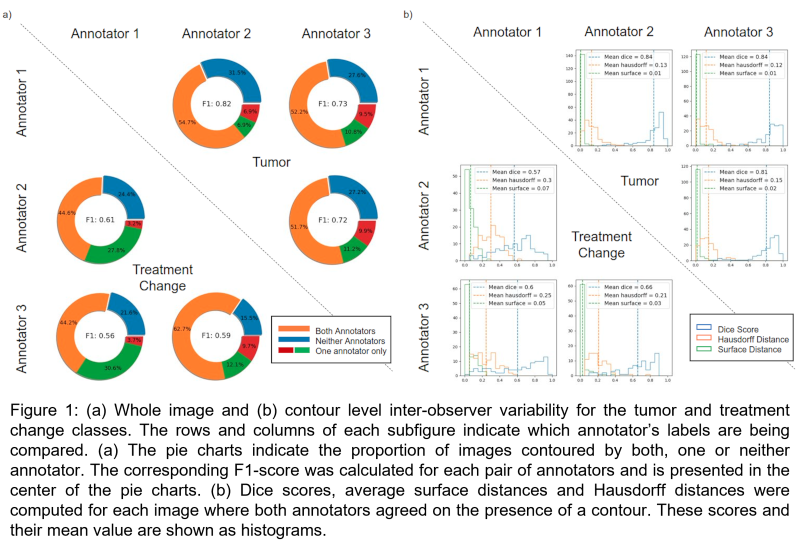
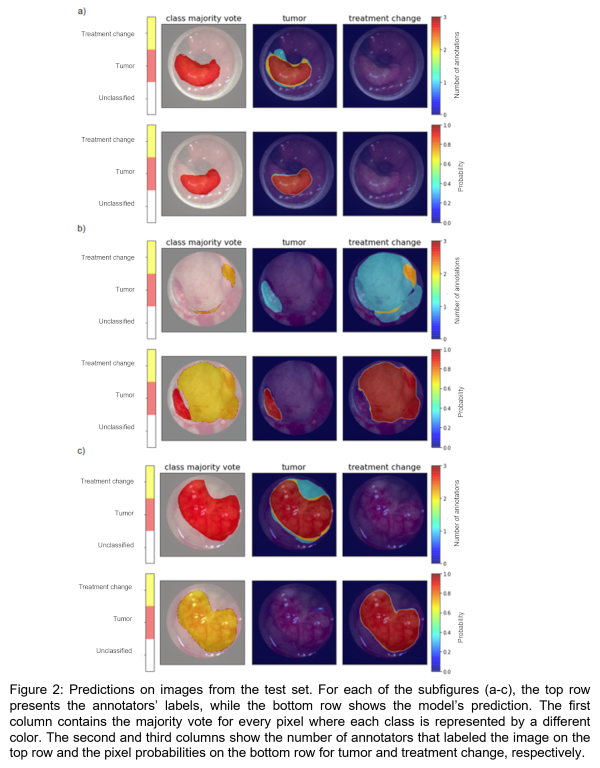
Conclusion
This study illustrated the important inter-observer variability associated with the task of segmenting endoscopy images. The results suggest that ground-truth data should be composed of labels from multiple annotators to avoid bias. Additional data are needed to increase the developed model’s performance and generalizability.