Commercial deep learning-based automated treatment planning validation for oropharyngeal cancer
Frank Dankers,
The Netherlands
PO-1631
Abstract
Commercial deep learning-based automated treatment planning validation for oropharyngeal cancer
Authors: Frank Dankers1, Peranavan Balasupramaniam2, Marije Brussee1, Alice Onderwater1, Martin de Jong1, Eleftheria Astreinidou1
1Leiden University Medical Center, Radiotherapy, Leiden, The Netherlands; 2VU University, Faculty of Science, Amsterdam, The Netherlands
Show Affiliations
Hide Affiliations
Purpose or Objective
Radiotherapy treatment planning for head and neck cancer (HNC) is a labor-intensive process which can take up to a day per patient. Additionally, plan quality is highly dependent on the experience of the treatment planner. RayStation 10B offers deep learning-based autoplanning (DLAP) functionality using a 3D convolutional neural network which is claimed to generate clinically acceptable and deliverable treatment plans within 15 minutes. In this study, the performance of RayStation DLAP for oropharyngeal cancer is investigated by carrying out a dosimetric comparison between DLAP plans and corresponding clinical treatment plans that were manually generated.
Material and Methods
The RayStation DLAP model was trained by RaySearch on 100 HNC plans that conformed to the DAHANCA guidelines. The model was iteratively tuned to our department’s requirements by RaySearch using a training set of 5 patients, and validated on a set of 14 patients (unseen by RaySearch). Final DLAP plans, after five tuning rounds, were dosimetrically compared to the corresponding 14 clinical plans. All patients had oropharyngeal cancer and received 54.25 Gy to the elective lymph nodes (dose level 1) and 70 Gy to the primary tumor (dose level 2). Comparison of DVH parameters was performed using boxplots for clinical and planning target volumes as well as relevant organs at risk. Statistical significance was tested using a two-tailed Wilcoxon signed-rank test at the 0.05 level.
Results
DLAP plan generation took less than 15 minutes per plan. Target coverage for both dose levels met the clinical constraints (V95% ≥ 98%). Target coverage of the elective nodes increased significantly for the DLAP plans when compared to the clinical plans, from CTV V98% = 99.2 (98.7-99.3) % in the clinical plans to 99.6 (99.2-99.8) % in the DLAP plans, and from PTV V95% = 98.0 (98.0-98.1) % to 98.3 (98.2-98.4) % (see Figure 2). Target coverage of the tumor showed less variation in the DLAP plans (not significant). The mean dose for both target volumes increased significantly in the DLAP plans (median increase of 0.5% for both target volumes). OAR dose was either similar or decreased for the DLAP plans. Statistical significant decrease was observed for the glottic area, larynx supraglottis, and inferior and superior pharyngeal constrictor muscles (median decreases of 6.7 Gy, 7.2 Gy, 3.5 Gy, and 1.2 Gy respectively). In general, less variation was observed for most DVH parameters in the DLAP plans when compared to the clinical plans.
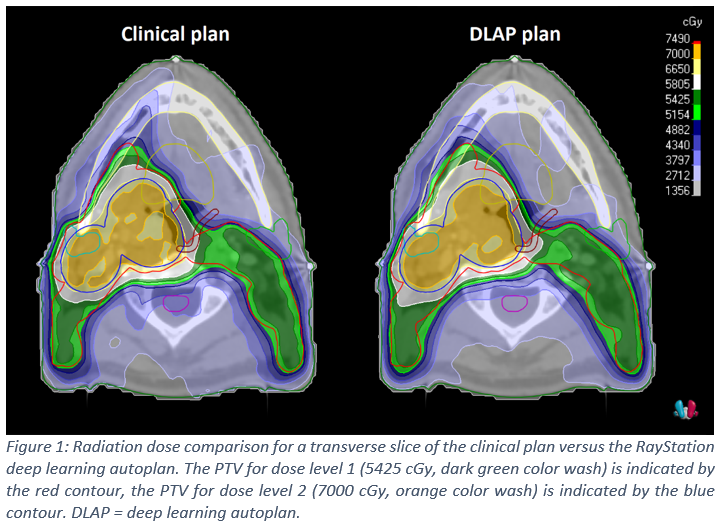
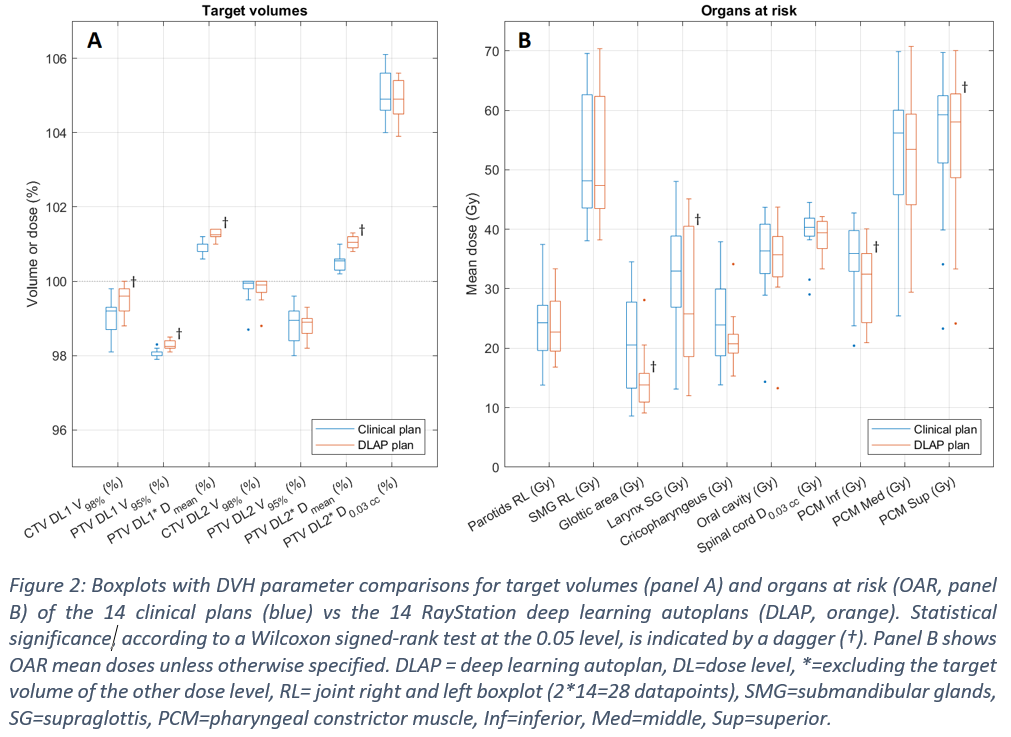
Conclusion
RayStation DLAP plans were compared to clinical treatment plans for 14 oropharyngeal cancer patients. Based on target volume and OAR DVH parameters, the DLAP plans either performed similar or improved significantly when compared to the clinical treatment plans. Additionally, DLAP showed less variation and hence improved treatment plan consistency over patients. We conclude that DLAP for oropharyngeal cancer patients should be considered for clinical introduction.