AI-based classifier using geometrical features to minimize heart dose in left breast with VMAT
PO-1619
Abstract
AI-based classifier using geometrical features to minimize heart dose in left breast with VMAT
Authors: Andrea Bresolin1, Pasqualina Gallo1, Francesco La Fauci1, Francesca Lobefalo1, Lucia Paganini1, Giacomo Reggiori1,2, Marco Pelizzoli1, Sara Parabicoli1, Nicola Lambri1,2, Pietro Mancosu1, Ruggero Spoto1, Luca Dominici1, Davide Franceschini3, Marta Scorsetti1,2, Stefano Tomatis1
1IRCCS Humanitas Research Hospital, Radiotherapy and Radiosurgery Department, Milan, Italy; 2Humanitas University, Department of Biomedical Sciences, Milan, Italy; 3IRCCS Humanitas Research Hospital, Radiotherapy and Radiosurgery Department, Rozzano, Italy
Show Affiliations
Hide Affiliations
Purpose or Objective
Radiotherapy treatment plan of the left breast is critical because of heart proximity. Mean heart dose (MHD) correlates with late heart toxicity. In this study, geometrical features accounting for Organs at Risk (OARs) proximity and structures shape of the breast treatment region were quantified using the Expansion Intersection Histogram method (EIH). An AI predictive model was used to predict MHD from these features. The impact of predictive variables on the plan complexity was also evaluated.
Material and Methods
379 consecutive VMAT left breast cancer patients treated between 2019-2022 at our Institute have been considered. Dose prescription to the whole breast was 40.5 Gy in 15 fractions, and 48 Gy to the surgical bed in simultaneous integrated boost (SIB, 254 cases). Progressive target isotropic expansions and mapping of the corresponding volume intersection with OARs into the EIH graph were automatically extracted using an in-house script. Separation (S, min non zero overlap expansion) and Wrapping (W, mean EIH slope between S and S+3 cm) were extracted as potential MHD predictors along with the volume (V) of the involved structures. The use of auto-planning, deep inspiration breath hold (DIBH), and the number of arcs were considered as additional features. A backward stepwise linear regression analysis (pr=0.01) was performed with MHD as the dependent variable. A 3 Gy MHD threshold, already applied in our department as a warning level, was used to label a case as “high risk” for late complications. The classifier was validated by ROC analysis. Cases were randomly assigned in the train (50%) and test (50%) sets. A plan complexity index (MITOTAL) was calculated for all plans and used in place of MHD as dependent variable of the regression model to associate geometry to plan complexity. Significance was set below the 0.05 level.
Results
The output of the regression model is shown in table 1 for the training set. The classification results for all patients are reported in figure 1. Multivariate association between geometrical features and MHD was demonstrated, with higher predicted MHDs related to larger targets, wrapped around the heart. An increase of 0.38 Gy was found when more than 2 VMAT arcs were used for therapy. The distance S between target and OARs was an effective MHD mitigating factor, as well as ipsi-lateral lung volume. MHD was also reduced by 0.32 Gy using auto-planning. MITOTAL showed a direct association with target volume (p<0.01), with a significant increase when auto-planning was used (p=0.03), while a negative correlation with the number of arcs was found (p<0.01).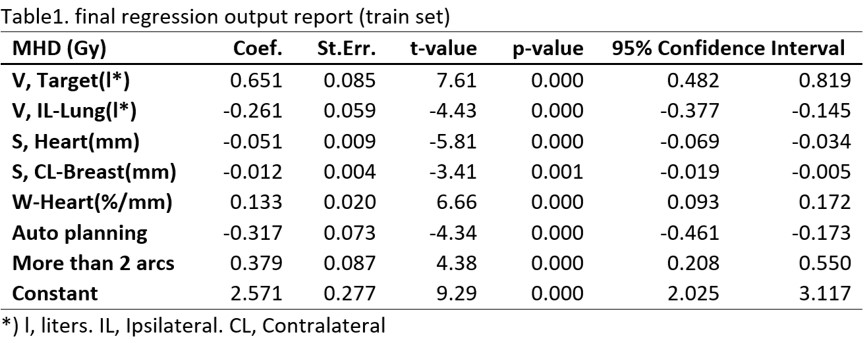
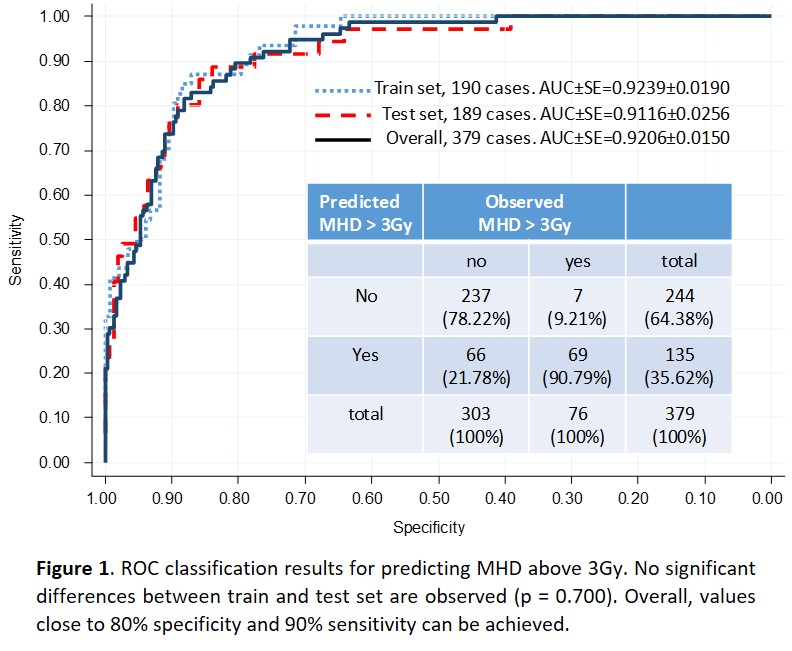
Conclusion
Data showed the ability of our AI model to predict MHD above 3Gy. This classifier may be used in early planning phase to choose the proper heart-sparing strategy. Although auto-planning reduced the MHD, this would increase the modulation.