Transferability of deep learning models to the segmentation of gross tumour volume in brain cancer
Emiliano Spezi,
United Kingdom
PO-1620
Abstract
Transferability of deep learning models to the segmentation of gross tumour volume in brain cancer
Authors: Abdulkerim Duman1, Philip Whybra1, James Powell2, Solly Thomas2, Xianfang Sun3, Emiliano Spezi1
1Cardiff University, School of Engineering, Cardiff, United Kingdom; 2Velindre NHS Trust, Department of Oncology, Cardiff, United Kingdom; 3Cardiff University, School of Computer Science and Informatics, Cardiff, United Kingdom
Show Affiliations
Hide Affiliations
Purpose or Objective
Automatic deep learning (DL) based tumour segmentation has the potential of reducing intra- and inter-observer variability. This could improve the quality of radiotherapy treatment plans. The accuracy of brain tumour delineation in radiation therapy planning is essential to deliver high doses to tumours while minimising radiation dose to the brain. Brain tumour segmentation is a complex task that can include different regions of the tumour such as: enhancing tumour (ET), tumour core (TC) and whole tumour (WT), as described in the brain tumour segmentation (BraTS) challenge [1]. In this work, we tested if deep learning models trained on the BraTS dataset could generate clinically useful Gross Tumour Volumes (GTVs) when applied to a local glioblastoma dataset.
Material and Methods
We developed a region-focused selection (RFS) method that combines several single 2D UNET [2] architectures trained on individual tumour regions and validated it performance using clinically defined GTVs as reference volumes. The BraTS 2021 training dataset (1251 patients with T1, T1ce, T2 and FLAIR MRI image sequences) and a local glioma dataset (53 patients with the same MRI sequences and clinically defined GTVs) were utilised. The DL segmentation models trained in this study used 2D UNET architectures. In the last layer of the UNET model, we applied a sigmoid function for both binary class and multilabel (overlapping class masks) approaches. For the multiclass (non-overlapping class masks) approach, the softmax function was implemented. Images were normalised using the z-score method. We trained multiclass, multilabel and binary class single 2D UNET architectures on individual tumour regions using the BraTS 2021 dataset. The Dice similarity coefficient (DSC) was used to evaluate the conformity of the contours generated by the DL models and reference contours. We then combined the best performing DL models into a union RFS (u-RFS) model. We tested all models for the segmentation of our local dataset and evaluated its performance against clinically defined GTVs.
Results
Table 1 shows that no single DL model outperforms the others for all regions. The multiclass segmentation approach had the best average DSC for ET (78.86%). The binary class approach performed the best average DSC for WT (84.80%). The multilabel approach obtained the best average DSC for TC (78.53%). u-RFS achieved the best average DSC for GTV (78.51%) segmentation. Figure 1 shows the best, average, and worst GTV segmentations for all models when applied to the local dataset.
Conclusion
This work shows that it is possible to transfer deep learning models trained on TC, to the segmentation of GTV in equivalent datasets. Our u-RFS model could generate clinically useful GTVs when applied to MRI images of glioblastoma.
References
[1] 10.1109/TMI.2014.2377694
[2] 10.1007/978-3-319-24574-4_28
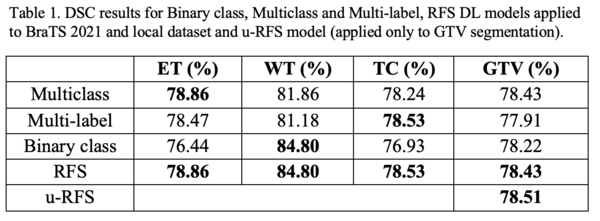
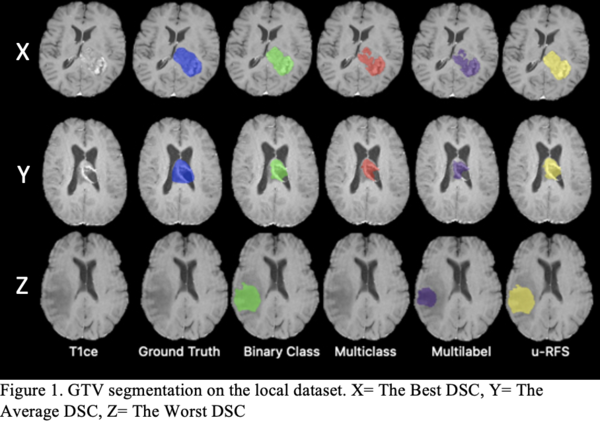