In vitro data selection strongly impact RBE model predictions
OC-0288
Abstract
In vitro data selection strongly impact RBE model predictions
Authors: Erlend Lyngholm1, Kristian S. Ytre-Hauge1, Camilla H. Stokkevåg1,2, Andreas H. Handeland1,2, Helge Henjum1, Johannes Tjelta3,2, Ilker Meric4, Armin Lühr5, Liheng Tian5
1University of Bergen, Department of Physics and Technology, Bergen, Norway; 2Haukeland University Hospital, Department of Oncology and Medical Physics, Bergen, Norway; 3University of Bergen, Department of Physics and Technology, Bergen, Norway; 4Western Norway University of Applied Sciences, Department of Computer science, Electrical engineering and Mathematical sciences, Bergen, Norway; 5TU Dortmund University, Department of Physics, Dortmund, Germany
Show Affiliations
Hide Affiliations
Purpose or Objective
While a constant relative biological effectiveness (RBE) of 1.1 still forms the basis for clinical proton treatment planning, RBE models are increasingly used in plan evaluation. There are however substantial variations between the RBE estimates across different models and several new in vitro datasets have been published but not yet accounted for in existing RBE models. Many models are based on data from monoenergetic (M) in vitro cell irradiation, while some also include data from spread out Bragg beak (SOBP) beams. The aim of this study was to identify changes in RBE trends compared to previously published models when analyzing all published proton in vitro RBE data. Specifically, it was investigated how database restrictions and differences between M and SOBP data affect RBE modelling.
Material and Methods
A proton database was collected from the literature. Each data point included LQ model parameters (α, β) and beam quality (LET) information. Data was fitted to RBE_max=1+(k∙LET)/(α⁄β)_x. Fits were performed with different database restrictions on the range of included LET and (α⁄β)_x values. The database was also fitted to previously published models. The models and refits were applied to predict biological doses along an SOBP for two different (α⁄β)_x values typical for early- and late responding tissue.
Results
The database contained 471 data points (196/275 M/SOBP), compared to 287 used for the most comprehensive published RBE model. The (α⁄β)_x distribution was dominated by values in the lower part of the range (Fig1A). The same was true for the LET values, but here the imbalance was mainly caused by the SOBP data (Fig1B). RBE_max (α/αx) and RBE_min (sqrt(β/βx)) also accumulate at lower values (Fig1C&D). Fitted k values were more affected by restrictions on LET values than those on (α⁄β)_x, especially for LET>20keV/μm (Fig1E). The fits to SOBP data consistently resulted in higher k than fits to M data. Applying models and refits in an SOBP scenario (Fig2A), if we only consider (α⁄β)_x dependent models (all except the Jones model), for low (α⁄β)_x the RBE estimate by refits and models (in parenthesis) was 1.15±0.08(1.10±0.03) in the entrance region, 1.20±0.06(1.18±0.08) in the middle of the SOBP and 1.32±0.11(1.33±0.18) in the distal part of the SOBP (Fig2G,E,C). Refitted (α⁄β)_x dependent models gave a narrower range of RBE estimates across models in the distal and middle part of the SOBP, but a wider range in the entrance region.
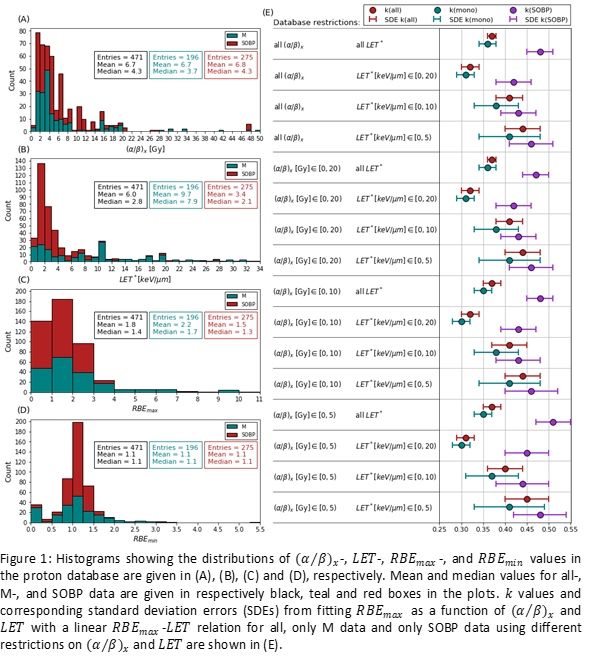
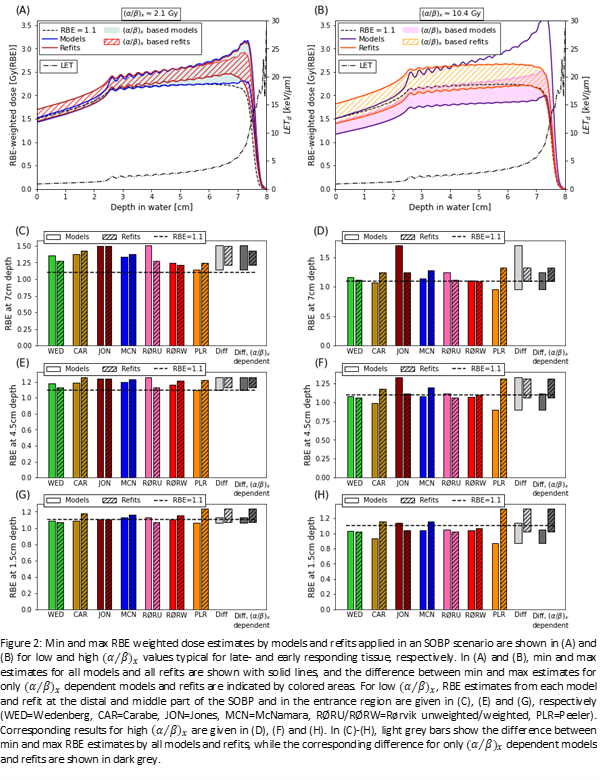
Conclusion
Database restrictions on LET had a greater effect on the fitting outcome than restrictions on (α⁄β)_x. SOBP data predicted steeper increase in RBE with increasing LET compared to M data. Refitting published RBE models to all available data had a relatively large impact on model estimates, indicating that the models might not generalize well when applied to data outside the databases used to develop them. For low (α⁄β)_x, where RBE variations are large, refits gave overall less RBE variations across the SOBP, especially in the distal part.