Full 3D CT reconstruction from partial bi-planar projections using a deep generative model
OC-0443
Abstract
Full 3D CT reconstruction from partial bi-planar projections using a deep generative model
Authors: Alexandre Cafaro1,2,3, Théophraste Henry3, Julie Colnot3, Quentin Spinat1, Amaury Leroy1,2,3, Pauline Maury3, Alexandre Munoz3, Guillaume Beldjoudi3, Ayoub Oumani1, Isabelle Chabert3, Léo Hardy1, Rafael Marini Silva1, Charlotte Robert3, Vincent Lepetit4, Nikos Paragios1, Eric Deutsch3,2, Vincent Grégoire3
1TheraPanacea, R&D Artificial Intelligence, Paris, France; 2Paris-Saclay University, Gustave Roussy, Inserm 1030, Molecular Radiotherapy and Therapeutic Innovation, Villejuif, France; 3Joint Collaboration Gustave Roussy - Centre Léon Bérard, Radiation Oncology, Villejuif-Lyon, France; 4Ecole des Ponts ParisTech, Research Artificial Intelligence, Marne-la-vallée, France
Show Affiliations
Hide Affiliations
Purpose or Objective
In radiation therapy (RT), patient positioning is achieved using images acquired either as multiple cone-beam kV projections or bi-planar orthogonal projections as in Exactrac or CyberKnife. The former is time-consuming and irradiates the patient over a large volume. It also lacks good attenuation for direct adaptive radiotherapy. The latter, less irradiant, is limited by its poor contrast and 2D nature. Both imaging systems also suffer from a small field of view (FOV), preventing replanning. In this context, we developed a deep generative neural network to reconstruct an extended 3D CT using only partial bi-planar synthetic projections. Our solution has the potential to drastically reduce time and imaging dose while allowing good tissue registration and adaptive radiotherapy.
Material and Methods
To ensure a good 3D reconstruction (RS) with only bi-planar projections, prior information must be leveraged. Thus, a 3D style-based generative network model (StyleGAN), was trained to learn the 3D distribution of head and neck CT structures, on a dataset of 3500 patients with cancer from 6 public cohorts and private internal data. Given a low-resolution signature, our StyleGAN generates a synthetic CT. Given 2 projections, we aim to find the signature and the position that generates the volume whose projections are the closest to the original ones. The latter are generated from CT elastically deformed on CBCT, i.e., a “virtual” CT (vCT). The out-of-field structure is completed by the pCT after rigid registration on the RS by deep learning. Our pipeline is described in Figure 1. We used 60 external patients undergoing VMAT treatment to benchmark the performance of our approach. Three RS metrics were calculated by comparison with the vCT and we compared our capacity of registering the pCT to our RS instead of the CBCT.
Results
Reconstruction of 3D volume from bi-planar projections takes only 30 seconds. Figure 2. shows examples of RSs. Our RS well fits the coarse patient structure with clear bone, tissue and air separation almost matching the vCT. We compute the similarity metrics between the RS and the vCT. On average, we achieve 2.26 % (std. 0.49 %) of RS error (RE), 26 dB of peak-signal-to-noise (PSNR) and 87% of structural similarity (SSIM). We also compare the translation and rotation parameters obtained to register the pCT to our RS with the clinical ones obtained with CBCT. On average we achieve a translation and rotation close by 0.4 mm, resp. 0.2° across all axes.
Conclusion
Our approach can reconstruct a full CT using only two partial biplanar images, with a quality almost on par with CBCT images while providing good tissue attenuation. It will accelerate on-board patient positioning and allow for adaptive radiotherapy. Future investigations will aim to make the reconstruction robust to real projections and increase the size of the reconstruction. Dosimetry simulations on our reconstructions will also be conducted to substantiate the impact for adaptive radiotherapy.
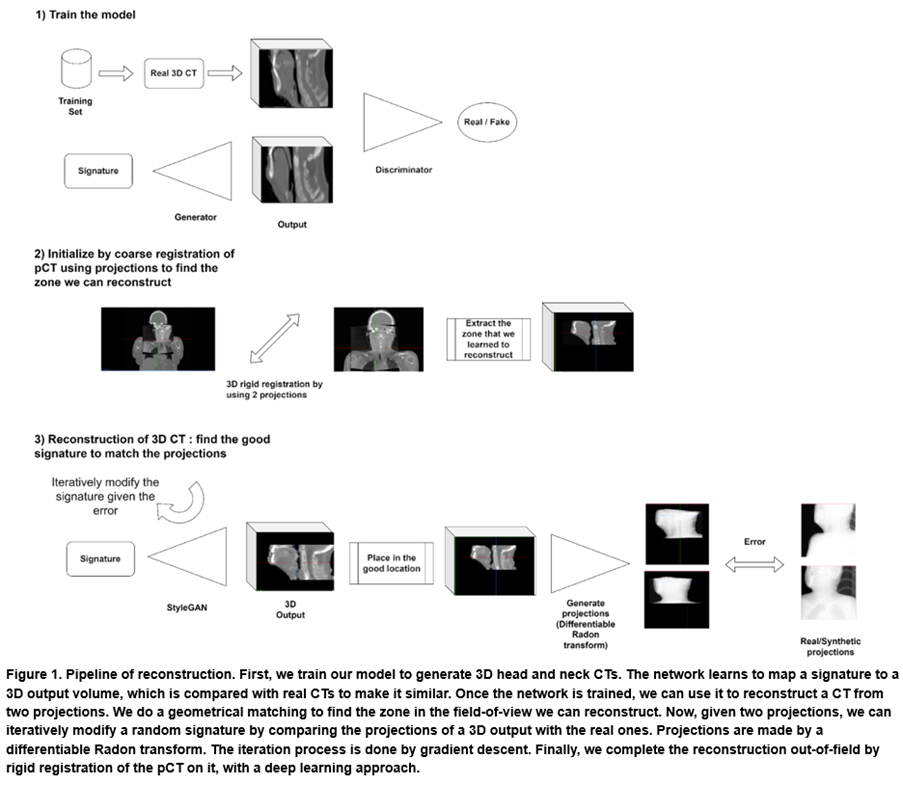
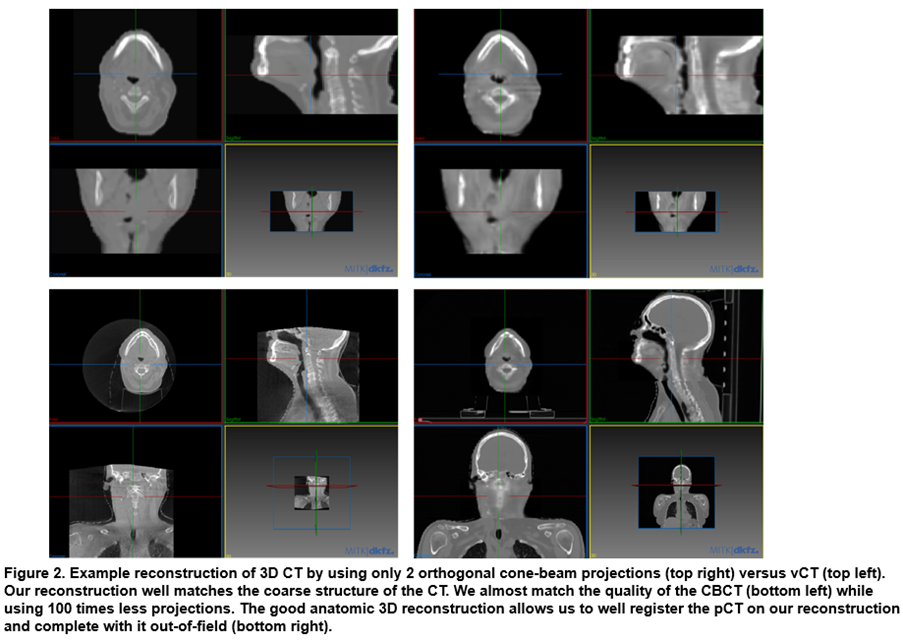