combining tumor and whole-lung radiomics features to predict prognosis in locally advanced NSCLC
Zhen Zhang,
The Netherlands
PD-0163
Abstract
combining tumor and whole-lung radiomics features to predict prognosis in locally advanced NSCLC
Authors: Zhen Zhang1, Meng Yan2, Zhixiang Wang1, Andre Dekker1, Leonard Wee1, Alberto Traverso1, Lujun Zhao2
1Maastricht University Medical Centre+, Department of Radiation Oncology (Maastro), Maastricht, The Netherlands; 2Tianjin Medical University Cancer Institute and Hospital, Department of Radiation Oncology, Tianjin, China
Show Affiliations
Hide Affiliations
Purpose or Objective
Several studies have shown that lung heterogeneity is associated with overall survival (OS) in lung cancer. However, the predictive value of whole lung-based radiomics for OS in non-small cell lung cancer (NSCLC) is unknown. The purpose of this study is to investigate the predictive value of whole lung-based and tumor-based radiomics for OS in locally advanced-NSCLC treated with curative radiotherapy.
Material and Methods
A total of 349 patients were enrolled in the study, with 292 patients in training set, 57 patients from the same hospital as independent test-set-1, and 47 patients from a multi-institutional prospective clinical trial data set (RTOG0617) as test-set-2. Tumor-based radiomics features and total lung-based radiomics features were extracted from planning CT images and radiomics features were selected by least absolute shrinkage embedded with Cox proportional hazards model with 5-fold cross-internal validation, with 1000 bootstrap samples. Radiomics prognostic scores (RS) were calculated by Cox regression based on selected features. Five models based on clinical factors, a tumor RS, and a lung RS separately and their combinations were constructed. The Harrell concordance index (C-index), calibration curves were used to evaluate the discrimination and calibration performance. Patients were stratified into high and low risk groups, and a log-rank test was performed.
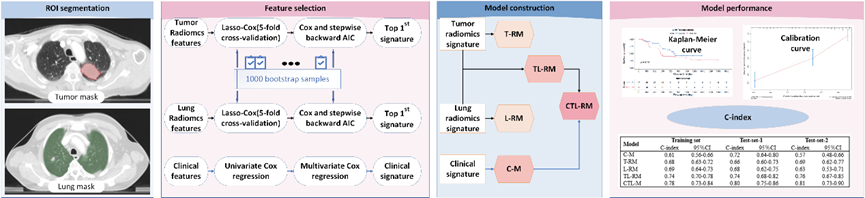
Figure 1: Workflow of the project. Abbreviations: L-RM: COX regression model based on lung signature; TL-RM: COX regression model based on tumor signature and lung signature; CTL-RM: COX regression model based on tumor signature, lung signature and clinical factors; C-index: Harrell concordance index.
Results
The discrimination ability of lung- and tumor-based radiomics model was similar in terms of C-index, 0.69 vs 0.68 in training set, 0.69 vs 0.66 in test-set-1, 0.63 vs 0.69 in test-set-2. The model that combined clinical factors, tumor and lung RS achieved the best performance (C-index, 0.78 in training set, 0.80 in test-set-1, 0.81 in test-set-2). The calibration curve showed good agreement between predicted and actual values. Patients were well stratified, with statistical significance for all datasets.

Figure 2: Kaplan-Meier survival curves based on the CTL-RM model in (A) training set, (B) test-set-1, (C) test-set-2. Abbreviations: CTL-RM: COX regression model based on tumor radiomics signature, lung radiomics signature and clinical factors.
Conclusion
Lung- and tumor-based radiomics features have the potential to predict OS in NSCLC. The combination of clinical and radiomics predictors can achieve optimal performance.