A Deep Learning multi-channel approach to abdominal synthetic CT for Carbon Ion Radiotherapy
PO-1951
Abstract
A Deep Learning multi-channel approach to abdominal synthetic CT for Carbon Ion Radiotherapy
Authors: Giovanni Parrella1, Alessandro Vai2, Anestis Nakas1, Noemi Garau1, Giorgia Meschini1, Silvia Molinelli2, Amelia Barcellini3, Andrea Pella4, Mario Ciocca5, Viviana Vitolo6, Ester Orlandi6, Chiara Paganelli1, Guido Baroni1
1Politecnico di Milano, Department of Electronics, Information and Bioengineering, Milano, Italy; 2National Center for Oncological Hadrontherapy, CNAO, Medical Physics unit, Pavia, Italy; 3National Center for Oncological Hadrontherapy, CNAO, Clinical Unit, Pavia, Italy; 4National Center for Oncological Hadrontherapy, CNAO, Bioengineering Unit, Pavia, Italy; 5National Center for Oncological Hadrontherapy, CNAO, Medical Physics, Pavia, Italy; 6National Center for Oncological Hadrontherapy, CNAO, Clinical Unit, Milano, Italy
Show Affiliations
Hide Affiliations
Purpose or Objective
MRI-guided radiotherapy is spreading thanks to the MRI great soft tissue contrast and the absence of additional ionizing radiations, but no integrated system is clinically available for particle therapy. Nonetheless, the off-line use of MRI may support treatment planning and adaptation through the generation of synthetic CT (sCT). In this context, Carbon Ions Radiotherapy (CIRT) rapresents a challenging field of application, due to the high sensitivity to inter and intra-fraction motion, especially for the abdominal district. Given the absence of literature studies that perform a dosimetric analysis on abdominal sCT applied to CIRT, we focused on a Deep Learning approach to abdominal sCTs, and its application in an MRI-only workflow for CIRT.
Material and Methods
39 abdominal MRI-CT volumes pairs were collected from 24 patients treated with CIRT at the National Centre for Oncological Hadrontherapy (CNAO). The sCTs were generated by a 3-channels (corresponding to Air, Bone and Soft tissue) conditional Generative Adversarial Network (cGAN) derived from the open source net “pix2pix”(Doi: 10.1109/CVPR.2017.632), trained with transversal slices (Figure 1). The hyperparameters of the net were tuned thorugh a 6-folds Cross Validation. The network was tested on 5 held-out patients in two frameworks: (i) a CT-based segmentation in Air, Bone, and Soft Tissues to assess the quality of sCT and (ii) a manual segmentation of MRI, to emulate an MRI-only scenario (MRI-only sCT).
The accuracy of sCTs was evaluated by means of similarity metrics (Mean Absolute Error, Normalized Cross Correlation, Structural Similarity Index and Peak Signal to Noise Ratio) and geometrical criteria (Dice Coefficient, Hausdorff Distance, Centre of Mass distance). The clinical CIRT plans were recalculated on MRI-only sCTs through a clinical TPS evaluated on the base of DVH comparison (D95% on GTV and CTV, D2% on OARs), global Gamma analysis (1mm/1%, 2mm/2%, 3mm/3%) and dose difference maps.
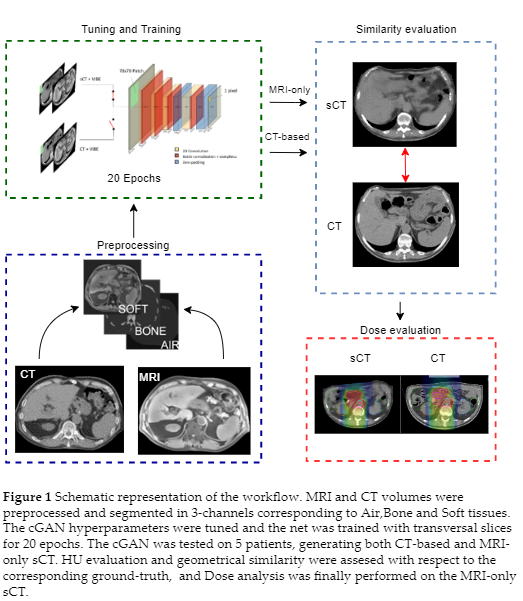
Results
All metrics for the CT-based test set were aligned with literature, with optimal MAE on bone structures (86.03 ± 10.76 HU) and soft tissues (55.39 ± 3.41 HU). The results on the MRI-only set were instead inferior (MAEBONE= 154.87 ± 22.90 HU) because of MRI manual segmentation and the lack of a proper ground truth, due to MRI-to-CT inter-acquisition motion. Despite the inconsistencies of air pockets, DVH metrics were comparable to literature (Figure 2, D95% GTV error = 1.88 ± 2.07 %), and the D2% relative error on OARs lay in an interquartile range of [-0.24,0.22]%. The air-filled organs showed the highest discrepancies, with colon being the most affected organ (relative error 12±16.41%), together with duodenum (1.89 ± 10.01%). Gamma pass rate reached 94.88 ± 4.9 % in case of 3%/3mm analysis.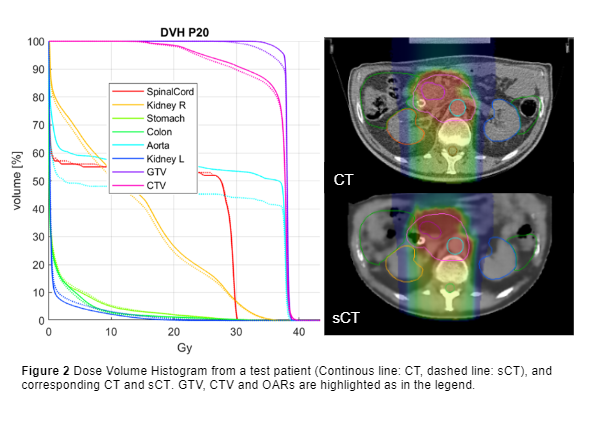
Conclusion
The proposed deep learning approach, based on a 3-channels cGAN, can generate good quality abdominal sCT, leading to promising CIRT dose recalculations.