Comparing LSTM networks for real-time target segmentation prediction on low-field MR-linacs
PO-1901
Abstract
Comparing LSTM networks for real-time target segmentation prediction on low-field MR-linacs
Authors: Elia Lombardo1, Moritz Rabe1, Yuqing Xiong1, Lukas Nierer1, Davide Cusumano2, Lorenzo Placidi2, Luca Boldrini2, Stefanie Corradini1, Maximilian Niyazi1, Michael Reiner1, Claus Belka1,3, Christopher Kurz1, Marco Riboldi4, Guillaume Landry1
1University Hospital, LMU Munich, Radiation Oncology, Munich, Germany; 2Fondazione Policlinico Universitario Agostino Gemelli, Radiation Oncology, Rome, Italy; 3German Cancer Consortium , DKTK, Munich, Germany; 4Ludwig-Maximilians-Universität München, Faculty of Physics, Munich, Germany
Show Affiliations
Hide Affiliations
Purpose or Objective
Respiratory motion leads to less conformal tumor treatment and increased dose to healthy tissues. Magnetic resonance imaging guided radiotherapy (MRgRT) enables the continuous acquisition of high soft tissue contrast images to monitor and potentially adapt to motion. Deformable multileaf collimator (MLC) tracking would allow to address both rigid displacement and deformation of the tumor without prolonging treatment times. However, to fully leverage deformable MLC tracking, the system latency must be accounted for by predicting future tumor contours in real-time. In this study, we compared the performance of two artificial intelligence (AI) algorithms based on long short-term memory (LSTM) modules for the prediction of 2D contours 500 ms into the future.
Material and Methods
The models were optimized and evaluated on contours extracted from sagittal 4 Hz cine MRs acquired during treatment with 0.35 T MR-linacs at two different institutions. Training and validation were based on 52 (3.1 h of motion) and 18 (0.6 h) patients treated at the University Hospital of the LMU Munich. For testing, we used additional 18 (1.1 h) patients from LMU and three (2.9 h) patients treated at Gemelli Hospital in Rome as a second testing set. Specifically, we implemented 1) a classical LSTM network (LSTM-shift), predicting future tumor centroid positions in superior-inferior and anterior-posterior direction which are used to shift the last observed tumor contour. The LSTM-shift model was optimized both in an offline and online fashion (offline and online LSTM-shift), the latter to enable patient-specific adaption to recent respiratory patterns. Furthermore, we implemented 2) a convolutional LSTM combined with spatial transformer layers (ConvLSTM-STL) to predict future deformation vector fields which are used to warp the last observed tumor contour. A no-predictor using the last available contour without any changes was implemented as baseline model. The performance of all models was evaluated with the Dice similarity coefficient, the 50% and the 95% Hausdorff distance (HD) between predicted and ground truth contours and the root mean squared error (RMSE) between the respective centroids.
Results
The online LSTM-shift performed the best for all computed metrics for both testing sets (Table 1). The ConvLSTM-STL achieved a better performance than the no-predictor, however, both were significantly worse than the online LSTM-shift. The online LSTM-shift achieved a 50% HD of 1.2 mm vs. 1.0 mm and a RMSE in superior-inferior direction of 1.3 mm vs. 1.1 mm for the LMU vs. Gemelli testing sets, respectively.
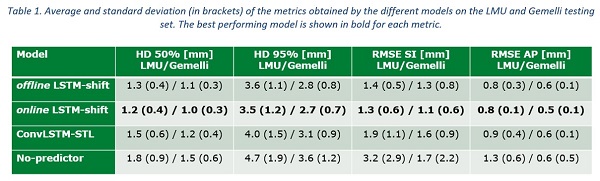
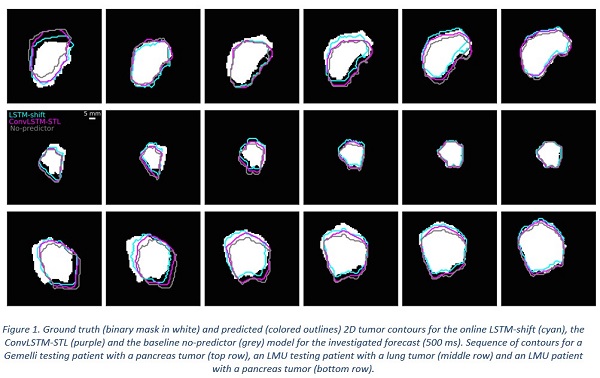
Conclusion
LSTM networks predicting centroid coordinates which are used to shift the last observed tumor contour appear to be the most suitable algorithms for contour prediction and could be used to compensate for the system latencies present in MRgRT with deformable MLC-tracking.