Editors’ pick
Optimal treatment plan adaptation using mid-treatment imaging biomarkers - PDF Version
Stefan CM ten Eikelder, Peter Ferjančič, Ali Ajdari, Thomas Bortfeld, Dick den Hertog and Robert Jeraj
Phys. Med. Biol. (2020). 65:245011. DOI: https://doi.org/10.1088/1361-6560/abc130.
What was your motivation for initiating this study?
A plethora of available biomarkers enables collection of patient-specific information before, during and after treatment. These markers can provide critical input into personalised treatment planning. With such information we face the question: how can this information be used to optimally adapt treatment? Little light has been shed on this question so far. Our work is the first step towards the framework entitled optimal stopping in radiation therapy (OSRT) [1]. The idea of OSRT is to view radiation therapy as a dynamic decision-making process. Based on the ‘state’ of the system (patient) and the available information at any ‘stage’ (fraction), the treatment is optimally adapted or stopped. In our paper, we have implemented one of the aspects of OSRT by adapting the dose optimally mid-treatment, with a special emphasis on information uncertainty. We have tested our methodology on a canine head-and-neck dataset, with the use of pre- and mid-treatment 3’-deoxy-3’[(18)F]-fluorothymidine (FLT) positron emission tomography/computed tomography ((PET/CT) scans as the biomarker for cell proliferation.
What were the main challenges during the work?
In order to adapt optimally to the mid-treatment imaging data, it is essential to establish a relationship between the imaging data and a quantifiable outcome measure. Initially, we considered incorporating data on clinical progression-free survival, but the dataset was too small to be used to construct a reliable model. Therefore, we decided to adopt the linear-quadratic Poisson tumour control probability (TCP) model, and use pre- and mid-treatment FLT PET scan data to estimate the model parameters (radiation sensitivity α and clonogen density). For the estimation, we made a few simple assumptions regarding early response. Naturally, the obtained parameter estimates were approximate. This posed an additional layer of uncertainty on top of the uncertainties in the data of the FLT PET images.
These uncertainties underline the need for robustness in optimal treatment adaptation strategies. We considered two adaptation strategies, both of which took uncertainties into account using a worst-case (i.e., min-max) approach. Uniform dose adaptation (UDA) scales the entire dose distribution upwards (dose escalation) or downwards (dose de-escalation), depending on the mid-treatment response observed from the imaging data. Continuous dose adaptation (CDA) involves re-optimisation of the fluence map, using normal tissue complication probability (NTCP) and TCP models, to redistribute dose according to spatial patterns of early treatment response.
What is the most important finding of your study?
The most important finding of our study is that we can expect improved benefit in TCP/NTCP for a substantial number of patients through employment of the OSRT paradigm, even in cases of relatively high uncertainties. For example, if we assume 10% uncertainty in radiation sensitivity and clonogen density estimates, the UDA and CDA adaptation strategies improve TCP intervals for four to six out of 14 patients, respectively, without noteworthy change in toxicity. Use of CDA leads to the ability to redistribute dose from radiosensitive voxels to radioresistant voxels, and often a clear visual distinction can be made between these sub-volumes.
There is currently no generally accepted method to determine the uncertainty level of response parameters that are derived from imaging data. However, our work provides insight into the reverse problem: what level of accuracy in parameter estimates is required for adaptations to be useful? Whereas at a 10% uncertainty level, treatment adaptation is clearly beneficial for several patients, the benefit of adaptation is unclear for most patients at 20%.
What are the implications of this research?
In our work we outline key components that are required for a treatment plan adaptation strategy that aims to optimise clinical benefit. Our strategy is not restricted to any specific biomarker or biological model. While we used FLT PET/CT imaging as a biomarker and TCP/NTCP models as biological response measures, one could use different biomarkers and different outcome models. However, the key point is that the components are sufficiently reliable; with too large uncertainties in biomarkers and/or biological response models, successful adaptation is not possible. In summary, our work helps to emphasise the importance of accounting for uncertainties in biologically-based adaptive treatment planning, both in the optimisation process itself and in the reporting of the results.
If you would like more information or wish to join the OSRT consortium, please visit:
https://gray.mgh.harvard.edu/research/osrt
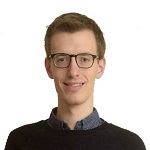
Stefan CM ten Eikelder
Department of Econometrics & Operations Research
Tilburg University
Tilburg, The Netherlands
s.c.m.teneikelder@tilburguniversity.edu
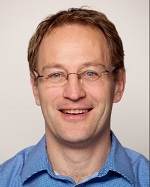
Robert Jeraj
Department of Medical Physics
University of Wisconsin - Madison
School of Medicine and Public Health
Madison, WI, USA
rjeraj@wisc.edu
[1] A. Ajdari, M. Niyazi, N. Nicolay, C. Thieke, R. Jeraj, T. Bortfeld (2019), Towards optimal stopping in radiation therapy, Radiotherapy and Oncology, 134: 96-100.