ESTRO 2024 Congress report
Why you should care about this work:
The calculation of accurate dose distributions is a critical step in radiotherapy planning, as the optimisation of the treatment plan as well as the estimation of expected tumour control and radiation-induced toxicity crucially depend on it. Currently, Monte-Carlo-based algorithms are considered gold standard for dose calculations, especially in strong magnetic fields, but their application is time-consuming due to their particle-wise simulation approach.
Innovations in radiotherapy, such as online adaptation that uses MRI, necessitate fast dose calculations to maximise the potential of online adaptive radiotherapy (OART). Delays in adaptation increase geometrical uncertainties and disrupt workflow efficiency.
Deep neural networks, known for modelling complex relationships quickly and accurately, offer a promising solution for fast-dose modelling in radiotherapy, enhancing OART further.
What we did:
We aimed to develop a deep neural network that was capable of modelling dose from single segments of a 1.5T MR-Linac treatment plan. As the approach is not per se based on fundamental radiation physics principles, a thorough evaluation was needed. Therefore, a broad clinical dataset that consisted of data from 125 MR-Linac patients, including information regarding prostate, liver, head-and-neck tumours, partial breast irradiations, and tumorous lymph nodes, was used. The dataset was split 60:40 between training and testing to ensure a comprehensive evaluation.
We implemented a 3D-Unet that utilised image data from planning CT and machine parameters as inputs, as proposed earlier [1]. The network was trained through the use of root-mean-squared error and a personalised loss function that emphasised high gradient areas in the dose domain. Full Monte-Carlo simulations served as ground truth [2]. The evaluation was conducted on both the clinical test dataset and in a standardised water phantom setting.
What we found:
The deep neural network accurately modelled dose distributions for all tumour entities (Figure 1). Despite not being optimised for maximum speed, the mean inference time for dose modelling was five seconds, compared with several hours for full Monte-Carlo simulations.
In the water phantom setting, the dose distribution was modelled accurately. This included correct dose build-up, dose decrease with water depth, and the electron return effect.
Regarding the clinical test dataset, high median gamma pass rates that measured the agreement between the modelled dose and ground truth were achieved. Depending on the tumour entity, median gamma pass rates for 3mm/3% distance-to-agreement ranged from 99.7% (prostate) to 96.7% (partial breast irradiation) for treatment plans, and from 99.4% (prostate) to 95.1% (tumorous lymph nodes) for individual irradiation segments. However, single-segment instances showed low accordance (minimum acceptance rate: 55%).
In conclusion, we demonstrated the strong potential of neural networks for dose modelling, which may be a powerful tool for fast dose calculation in future OART applications. Instances of low accordance highlight the need for comprehensive datasets, improved robustness, and built-in uncertainty estimation, which we plan to implement in the future.
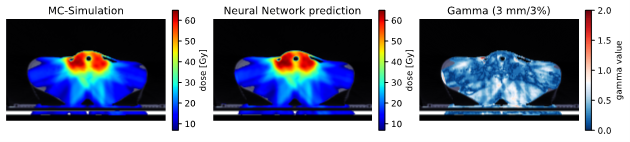

Figure 1: Isocentric slice of the Monte Carlo target dose distribution (left), the predicted dose distribution (middle) and the gamma map (3mm/3%, dose cutoff 10%, right) of a treatment plan from the head-and-neck test dataset (top) and a segment from the prostate test dataset (bottom). In the gamma map, values below or equal to 1.0 depict fulfilment of the criterion.
[1] Kontaxis C, Bol GH, Lagendijk JJW, Raaymakers BW. DeepDose: Towards a fast dose calculation engine for radiation therapy using deep learning. Phys Med Biol 2020;65:075013. https://doi.org/10.1088/1361-6560/ab7630.
[2] Friedel M, Nachbar M, Mönnich D, Dohm O, Thorwarth D. Development and validation of a 1.5 T MR‐Linac full accelerator head and cryostat model for Monte Carlo dose simulations. Med Phys 2019;46:5304–13. https://doi.org/10.1002/mp.13829.
Moritz Schneider

Section for Biomedical Physics, Department of Radiation Oncology, University Hospital Tübingen, Tübingen, Germany
moritz.schneider@med.uni-tuebingen.de