Editor's Pick
RapidBrachyDL: Rapid Radiation Dose Calculations in Brachytherapy via Deep Learning - PDF Version
Mao X, Pineau J, Keyes R, Enger SA.
Int J Radiat Oncol Biol Phys. 2020 Nov 1;108(3):802-812.
What was your motivation for initiating this study?
In recent years, brachytherapy has undergone a renaissance with enhanced use of magnetic-resonance-based imaging and treatment planning, 3D-printed personalised applicators, inverse dose optimisation and innovations such as intensity-modulated brachytherapy with partially shielded applicators1-3. Unfortunately, clinical treatment planning software (TPS) are still based on the water kernel superposition algorithm recommended by the American Association of Physicists in Medicine’s (AAPM) Task Group (TG) 43U14. This formalism assumes that the patient’s body is an infinite water phantom of uniform density. The influence of tissue, applicator material and shield heterogeneities, intersource attenuation and finite patient dimensions are ignored.5 In addition, only vendor-specific applicators and technology are supported by clinical TPS. Due to these limitations, the use of accurate dosimetry and innovations in brachytherapy such as novel brachytherapy applicators and delivery systems is challenging.
Accurate dose calculations can be achieved with model-based dose calculation algorithms (MBDCAs); the Monte Carlo method is the gold standard. In 2012, the AAPM released the findings of TG186, which advocated the use of MBDCAs.6 Currently, there are two separate MBDCA-based commercial TPS: the Oncentra advanced collapsed-cone engine, which was released as part of the Oncentra Brachy TPS (Elekta Brachytherapy, Veenendaal, Netherlands) and Acuros, which is incorporated in the BrachyVision TPS (Varian Medical Systems, Palo Alto, CA). Clinical MBDCAs have more limitations than the Monte Carlo method, and, again, users are limited to vendor-specific applicator libraries and prefixed calculation settings that meet vendor optimisation schemes.7
To support the development of accurate, patient-specific dosimetry and innovations in brachytherapy, our group released an open-source Monte Carlo-based TPS called RapidBrachyMCTPS8,9. However, the Monte Carlo method can be slow for use in time-sensitive clinical workflow. The motivation for this study was to replace the Monte Carlo-based dose calculation engine in RapidBrachyMCTPS with a three-dimensional (3D) deep convolutional neural network (CNN) model called RapidBrachyDL to provide fast and accurate dose estimations.10
What are the most important findings of your study?
If RapidBrachyDL is given treatment plans and patient geometries, it predicts Monte Carlo-calculated dose maps. It consists of three main components, with both input and output data in 3D format. The first component is a simple CNN that is responsible for the prediction of dose to water. This CNN is trained during the pretraining phase and is fixed afterwards. The second and third components work jointly to produce the final dose predictions in the medium, and their intended functions are to provide modifications to the output of the first component. More specifically, the second component is a feature-wise network based on linear modulation11, which is in charge of providing the conditioning, and the third part is a 3D network based on U-net12, which handles the generation of the main dose to the medium.
RapidBrachyDL models the underlying physics of the interaction of radiation with matter, rather than the standard first-principles, physics-based methods for calculation of absorbed dose. Hence it provides a solution to the accuracy-time trade-off that occurs in traditional Monte Carlo calculations. Since RapidBrachyDL uses gold-standard Monte Carlo simulations to generate training data and then uses the data to optimise its own parameters, it can calculate radiation quantities quickly at accuracies that are arbitrarily close to those of the source Monte Carlo algorithm, but with much faster computation times.
What were the main challenges during the work?
To obtain a model suitable for general-propose brachytherapy dosimetry, a large amount of diverse data is required, in terms of tumour sites, different tissue types and applicator materials, shielded applicators, and different radiation sources. Extensive Monte Carlo simulations are currently ongoing to create ground-truth data for all tumour types that are treated through brachytherapy and all clinical/hypothetical radiation sources that are used in treatments, in order to train RapidBrachyDL and to make it a general-purpose dose estimation engine for brachytherapy applications.
What are the implications of this research?
RapidBrachyDL has made it possible to obtain accurate, patient-specific doses in a clinical workflow on a personal computer or laptop, in seconds.
.jpg.aspx)
Shirin A. Enger, PhD
Co-director (interim), Medical Physics Unit
Canada research chair in medical physics
Associate professor, Gerald Bronfman Department of Oncology
Associate member, Department of Physics
Department of Biomedical Engineering
McGill University; and
Research director in translational physics and radiation biology
Lady Davis Institute and Segal Cancer Centre
Jewish General Hospital
Montréal, Québec, Canada
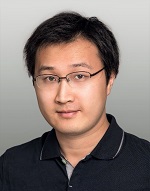
Ximeng Mao
Department of Computer Science
Medical Physics Unit
McGill University
Montréal, Québec, Canada; and
Mila-Quebec Artificial Intelligence Institute
Montréal, Québec, Canada
References
- Callaghan CM, Adams Q, Flynn RT, Wu X, Xu W, Kim Y. Systematic Review of Intensity-Modulated Brachytherapy (IMBT): Static and Dynamic Techniques. Int J Radiat Oncol. 2019;105(1):206-221.
- Famulari G, Duclos M, Enger SA. A novel 169Yb-based dynamic-shield intensity modulated brachytherapy delivery system for prostate cancer. Med Phys. 2020;47(3):859-868.
- Morcos M, Antaki M, Viswanathan AN, Enger SA. A novel minimally invasive dynamic-shield, intensity-modulated brachytherapy system for the treatment of cervical cancer. Med Phys. 2021;48(1):71-79.
- Rivard MJ, Coursey BM, DeWerd LA, Hanson WF, Saiful Huq M, Ibbott GS, Mitch MG, Nath R, Williamson JF. Update of AAPM Task Group No. 43 Report: A revised AAPM protocol for brachytherapy dose calculations. Med Phys. 2004;31(3):633–74.
- Enger SA, Vijande J, Rivard MJ. Model-Based Dose Calculation Algorithms for Brachytherapy Dosimetry. Semin Radiat Oncol. 2020;30(1):77-86.
- Beaulieu L, Carlsson Tedgren Å, Carrier JF, Davis SD, Mourtada F, Rivard MJ, Thomson RM, Verhaegen F, Wareing TA, Williamson JF. Report of the Task Group 186 on model-based dose calculation methods in brachytherapy beyond the TG-43 formalism: Current status and recommendations for clinical implementation. Med Phys. 2012;39(10):6208–36.
- Papagiannis P, Pantelis E, Karaiskos P. Current state of the art brachytherapy treatment planning dosimetry algorithms. Br J Radiol 2014; 87:20140163.
- Famulari G, Renaud MA, Poole C, Evans M, Seuntjens J, Enger SA. RapidBrachyMCTPS: a Monte Carlo-based treatment planning system for brachytherapy applications. Phys Med Biol. 2018; 63:175007.
- Glickman H, Antaki M, Deufel C, Enger SA. RapidBrachyMCTPS 2.0: A Comprehensive and Flexible Monte Carlo-Based Treatment Planning System for Brachytherapy Applications. arXiv Prepr arXiv200702902. Published online 2020.
- Mao X, Pineau J, Keyes R, Enger SA. RapidBrachyDL: Rapid Radiation Dose Calculations in Brachytherapy Via Deep Learning. Int J Radiat Oncol Biol Phys. 2020;108(3):802-812.
- Perez E, Strub F, Vries HD, Dumoulin V, Courville AC. Film: Visual reasoning with a general conditioning layer. Association for the Advancement of Artificial Intelligence Conference on Artificial Intelligence (AAAI). 2018;3942-3951.
- Çiçek Ö, Abdulkadir A, Lienkamp S, Brox T, Ronneberger O. 3D U-Net: Learning Dense Volumetric Segmentation from Sparse Annotation. Medical Image Computing and Computer-Assisted Intervention (MICCAI). 2016; 9901:424-432.